Samuel Blau - High-Throughput DFT and Monte Carlo for Reaction Networks and Machine Learning
Recorded 01 May 2023. Samuel Blau of Lawrence Berkeley National Laboratory presents "High-Throughput DFT and Monte Carlo for Reaction Networks and Machine Learning" at IPAM's workshop for Complex Scientific Workflows at Extreme Computational Scales.
Abstract: The Blau group uses high-throughput calculations to enable data-driven investigation and machine learning of complex chemical systems. Our self-correcting molecular DFT workflows allow for the simulation of thousands of charged and open-shell species for the first time. This allows us to construct and analyze massive filter-based chemical reaction networks describing electron-driven reaction cascades in both battery electrolytes and extreme ultraviolet lithography, two applications where chemical mechanisms are poorly understood and novel insight is critical to advancing energy technologies. We further leverage high-performance kinetic Monte Carlo simulations both to analyze these reaction networks and to simulate the photophysical dynamics of upconverting nanoparticles (UCNPs). We have generated the first ever large dataset of over 5000 simulated UCNP emissions spectra, and we use this data to train a novel graph-based machine learning model that predicts spectra given layer thicknesses and dopant concentrations. Automatic differentiation of our model enables inverse design of nanoparticle heterostructures in order to maximize UV emission for bioimaging and 3D printing.
Learn more online at: hhttp://www.ipam.ucla.edu/programs/workshops/workshop-iii-complex-scientific-workflows-at-extreme-computational-scales/
Видео Samuel Blau - High-Throughput DFT and Monte Carlo for Reaction Networks and Machine Learning канала Institute for Pure & Applied Mathematics (IPAM)
Abstract: The Blau group uses high-throughput calculations to enable data-driven investigation and machine learning of complex chemical systems. Our self-correcting molecular DFT workflows allow for the simulation of thousands of charged and open-shell species for the first time. This allows us to construct and analyze massive filter-based chemical reaction networks describing electron-driven reaction cascades in both battery electrolytes and extreme ultraviolet lithography, two applications where chemical mechanisms are poorly understood and novel insight is critical to advancing energy technologies. We further leverage high-performance kinetic Monte Carlo simulations both to analyze these reaction networks and to simulate the photophysical dynamics of upconverting nanoparticles (UCNPs). We have generated the first ever large dataset of over 5000 simulated UCNP emissions spectra, and we use this data to train a novel graph-based machine learning model that predicts spectra given layer thicknesses and dopant concentrations. Automatic differentiation of our model enables inverse design of nanoparticle heterostructures in order to maximize UV emission for bioimaging and 3D printing.
Learn more online at: hhttp://www.ipam.ucla.edu/programs/workshops/workshop-iii-complex-scientific-workflows-at-extreme-computational-scales/
Видео Samuel Blau - High-Throughput DFT and Monte Carlo for Reaction Networks and Machine Learning канала Institute for Pure & Applied Mathematics (IPAM)
Показать
Комментарии отсутствуют
Информация о видео
2 мая 2023 г. 5:35:16
00:50:19
Другие видео канала
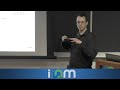
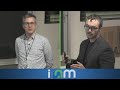
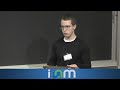
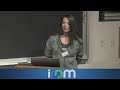
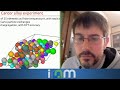
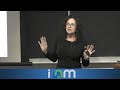
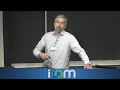
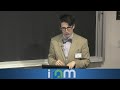
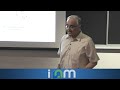
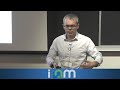
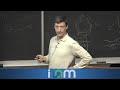
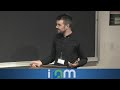
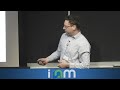
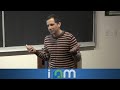
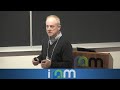
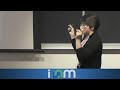
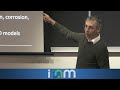
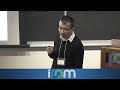
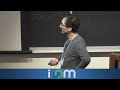
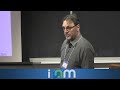