Joshua Schrier - Creating Complex Scientific Workflows that Reach into the Real World - IPAM at UCLA
Recorded 02 May 2023. Joshua Schrier of Fordham University presents "Creating Complex Scientific Workflows that Reach into the Real World" at IPAM's workshop for Complex Scientific Workflows at Extreme Computational Scales.
Abstract: A growing field of laboratory automation attempts to give computational workflows "hands" and "eyes" in the laboratory. Achieving this goal requires specifying unambiguous machine-readable experiments plans, capturing comprehensive data and metadata during experiments, and processing the collected data into a form suitable for machine-learning. While one platonic ideal would have a completely autonomous ("closed loop" or "self-driving") experimental workflow, in practice, most experiments involve islands of automation with varying amounts of sample preparation done by human technicians who must also be instructed and given an opportunity to capture data.
In this talk, I will talk about our experience in developing the open-source ESCALATE (Experiment Specification, Capture And Laboratory Automation TEchnology) data management software. The core motivation was to create an abstraction layer for experiment planning algorithms that abstracts away the details of how to tell the humans and machines what to do and capture and interpret those results. We've tested this in the context of distributed, partially-automated syntheses of halide perovskites. To facilitate machine learning, we include packages for automatically adding cheminformatics descriptor featurizations to the data. Interaction can occur either through a web-based GUI or through a REST-API, allowing for both human and computer-based experiment specification and data abstraction. We've tested this on several projects, including a distributed "bakeoff competition" in which third-party participants competed in directing new exploratory synthesis experiments. I will conclude by reflecting on the challenges of developing and maintaining software like this in small academic groups.
Learn more online at: hhttp://www.ipam.ucla.edu/programs/workshops/workshop-iii-complex-scientific-workflows-at-extreme-computational-scales/
Видео Joshua Schrier - Creating Complex Scientific Workflows that Reach into the Real World - IPAM at UCLA канала Institute for Pure & Applied Mathematics (IPAM)
Abstract: A growing field of laboratory automation attempts to give computational workflows "hands" and "eyes" in the laboratory. Achieving this goal requires specifying unambiguous machine-readable experiments plans, capturing comprehensive data and metadata during experiments, and processing the collected data into a form suitable for machine-learning. While one platonic ideal would have a completely autonomous ("closed loop" or "self-driving") experimental workflow, in practice, most experiments involve islands of automation with varying amounts of sample preparation done by human technicians who must also be instructed and given an opportunity to capture data.
In this talk, I will talk about our experience in developing the open-source ESCALATE (Experiment Specification, Capture And Laboratory Automation TEchnology) data management software. The core motivation was to create an abstraction layer for experiment planning algorithms that abstracts away the details of how to tell the humans and machines what to do and capture and interpret those results. We've tested this in the context of distributed, partially-automated syntheses of halide perovskites. To facilitate machine learning, we include packages for automatically adding cheminformatics descriptor featurizations to the data. Interaction can occur either through a web-based GUI or through a REST-API, allowing for both human and computer-based experiment specification and data abstraction. We've tested this on several projects, including a distributed "bakeoff competition" in which third-party participants competed in directing new exploratory synthesis experiments. I will conclude by reflecting on the challenges of developing and maintaining software like this in small academic groups.
Learn more online at: hhttp://www.ipam.ucla.edu/programs/workshops/workshop-iii-complex-scientific-workflows-at-extreme-computational-scales/
Видео Joshua Schrier - Creating Complex Scientific Workflows that Reach into the Real World - IPAM at UCLA канала Institute for Pure & Applied Mathematics (IPAM)
Показать
Комментарии отсутствуют
Информация о видео
3 мая 2023 г. 6:27:23
00:48:36
Другие видео канала
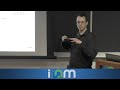
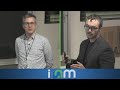
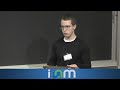
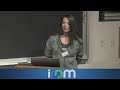
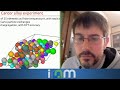
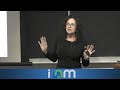
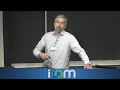
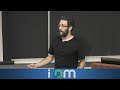
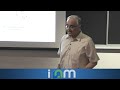
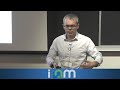
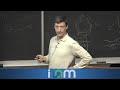
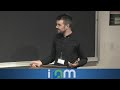
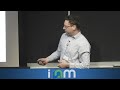
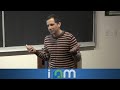
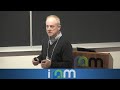
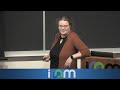
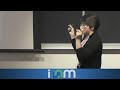
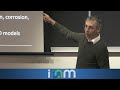
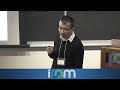
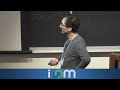