Amit Acharya - Slow time-scale behavior of fast microscopic dynamics - IPAM at UCLA
Recorded 21 April 2023. Amit Acharya of Carnegie Mellon University presents "Slow time-scale behavior of fast microscopic dynamics" at IPAM's workshop for Scale-Bridging Materials Modeling at Extreme Computational Scales.
Abstract: This talk aims to understand and exploit the slow time-scale behavior of rapidly evolving microscopic dynamics posed in terms of systems of non-linear ordinary differential equations, not necessarily containing an a priori split into fast and slow variables. Such a question arises naturally and ubiquitously in efforts to understand macroscopic dynamics, on engineering time-scales, of well-accepted models of microscopic dynamics that, however, are not amenable to practical computing over the much, much larger macroscopic time-scales of interest. This is because there is a vast separation of time scales involved between the dynamics of the macroscopic variables of interest and the microscopic dynamics, and evolving the microscopic dynamics directly fails to address the question of the macroscopic dynamics. The methodology employed involves a computational scheme based on fundamental mathematical theory that a) defines appropriate `coarse' variables corresponding to the microscopic dynamics that evolve in a stable manner on the coarse time scale; b) determines the equation of evolution for such variables; and c) defines a practically useful strategy for accurately initializing short bursts of microscopic runs for the evolution of the slow variables, without special requirements on the nature of the microscopic dynamics. We will illustrate the theory with examples that violate ergodicity and include both conservative and dissipative behavior. A first step towards coarse graining of discrete dislocation dynamics to a pde-based plasticity model without relying on constitutive assumptions will also be demonstrated.
This is joint work with Sabyasachi Chatterjee, Zvi Artstein, Giacomo Po, Xiaohan Zhang, and Nasr Ghoniem.
Learn more online at: http://www.ipam.ucla.edu/programs/workshops/workshop-ii-scale-bridging-materials-modeling-at-extreme-computational-scales/
Видео Amit Acharya - Slow time-scale behavior of fast microscopic dynamics - IPAM at UCLA канала Institute for Pure & Applied Mathematics (IPAM)
Abstract: This talk aims to understand and exploit the slow time-scale behavior of rapidly evolving microscopic dynamics posed in terms of systems of non-linear ordinary differential equations, not necessarily containing an a priori split into fast and slow variables. Such a question arises naturally and ubiquitously in efforts to understand macroscopic dynamics, on engineering time-scales, of well-accepted models of microscopic dynamics that, however, are not amenable to practical computing over the much, much larger macroscopic time-scales of interest. This is because there is a vast separation of time scales involved between the dynamics of the macroscopic variables of interest and the microscopic dynamics, and evolving the microscopic dynamics directly fails to address the question of the macroscopic dynamics. The methodology employed involves a computational scheme based on fundamental mathematical theory that a) defines appropriate `coarse' variables corresponding to the microscopic dynamics that evolve in a stable manner on the coarse time scale; b) determines the equation of evolution for such variables; and c) defines a practically useful strategy for accurately initializing short bursts of microscopic runs for the evolution of the slow variables, without special requirements on the nature of the microscopic dynamics. We will illustrate the theory with examples that violate ergodicity and include both conservative and dissipative behavior. A first step towards coarse graining of discrete dislocation dynamics to a pde-based plasticity model without relying on constitutive assumptions will also be demonstrated.
This is joint work with Sabyasachi Chatterjee, Zvi Artstein, Giacomo Po, Xiaohan Zhang, and Nasr Ghoniem.
Learn more online at: http://www.ipam.ucla.edu/programs/workshops/workshop-ii-scale-bridging-materials-modeling-at-extreme-computational-scales/
Видео Amit Acharya - Slow time-scale behavior of fast microscopic dynamics - IPAM at UCLA канала Institute for Pure & Applied Mathematics (IPAM)
Показать
Комментарии отсутствуют
Информация о видео
22 апреля 2023 г. 2:43:29
00:55:57
Другие видео канала
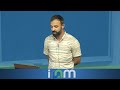
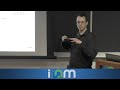
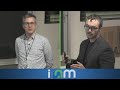
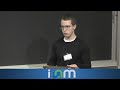
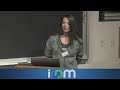
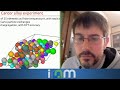
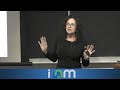
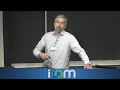
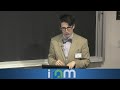
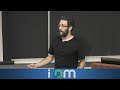
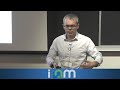
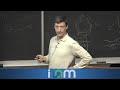
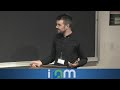
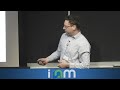
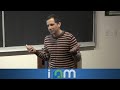
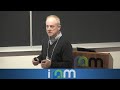
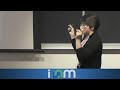
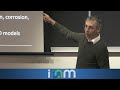
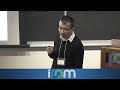
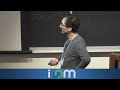