Richard Hennig & Jason Gibson - AI-driven workflows for the discovery of novel superconductors
Recorded 05 May 2023. Richard Hennig and Jason Gibson of the University of Florida present "AI-driven workflows for the discovery of novel superconductors" at IPAM's workshop for Complex Scientific Workflows at Extreme Computational Scales.
Abstract: Over the past decade, ab-initio structure prediction methods and artificial intelligence-based screening approaches resulted in many new materials and significantly impacted discovery and design. This progress is due to advances in computing power and algorithms. Further accelerating the search for new materials and utilizing available data requires complex AI workflows that identify favorable candidate structures, determine their stability, and rapidly predict phase diagrams for synthesis and materials properties for applications. This talk will discuss three AI algorithms for workflows that aim to identify novel superconductors. First, data augmentation by structural perturbation improves the fidelity of crystal graph neural networks trained on large datasets of relaxed to predict the relaxed formation energies of unrelaxed structures [1]. This augmentation improves predictions for unrelaxed structures by 66%. Second, we combine effective many-body potentials in a cubic B-spline basis with regularized linear regression to obtain machine-learning potentials that are physically interpretable, sufficiently accurate for applications, and as fast as the fastest traditional empirical potentials [2]. We show that these ultra-fast potentials are two to four orders of magnitude faster than state-of-the-art machine-learning potentials but close in accuracy and enable rapid relaxations and dynamics simulations of large atomistic systems. Finally, we show that symbolic regression methods coupled with physical insights can discover empirical relations and improve previous equations, such as the Allen-Dynes equation for predictions of superconducting transition temperatures based on the electron-phonon spectral function [3,4]. These three methods demonstrate the power of machine learning to accelerate the discovery and design of materials and illustrate some of the issues for developing efficient complex workflows for materials.
[1] Data-augmentation for graph neural network learning of the relaxed energies of unrelaxed structures.
J. Gibson, A. C. Hire, and R. G. Hennig, npj Computational Materials 8, 2115 (2022), doi:10.1038/s41524-022-00891-8.
[2] Ultra-fast interpretable machine-learning potentials. S. R. Xie, M. Rupp, and R. G. Hennig, arXiv:2110.00624 (2020), doi:10.48550/arXiv.2110.00624.
[3] Machine learning of superconducting critical temperature from Eliashberg theory. S. R. Xie, Y. Quan, A. C. Hire, B. Deng, J. M. DeStefano, I. Salinas, U. S. Shah, L. Fanfarillo, J. Lim, J. Kim, G. R. Stewart, J. J. Hamlin, P. J. Hirschfeld, and R. G. Hennig, npj Computational Materials 8, 1 (2022), doi:10.1038/s41524-021-00666-7.
[4] Functional Form of the Superconducting Critical Temperature from Machine Learning. S. R. Xie, G. R. Stewart, J. J. Hamlin, P. J. Hirschfeld, and R. G. Hennig, Phys. Rev. B 100, 174513 (2019), doi:10.1103/PhysRevB.100.174513.
Learn more online at: hhttp://www.ipam.ucla.edu/programs/workshops/workshop-iii-complex-scientific-workflows-at-extreme-computational-scales/
Видео Richard Hennig & Jason Gibson - AI-driven workflows for the discovery of novel superconductors канала Institute for Pure & Applied Mathematics (IPAM)
Abstract: Over the past decade, ab-initio structure prediction methods and artificial intelligence-based screening approaches resulted in many new materials and significantly impacted discovery and design. This progress is due to advances in computing power and algorithms. Further accelerating the search for new materials and utilizing available data requires complex AI workflows that identify favorable candidate structures, determine their stability, and rapidly predict phase diagrams for synthesis and materials properties for applications. This talk will discuss three AI algorithms for workflows that aim to identify novel superconductors. First, data augmentation by structural perturbation improves the fidelity of crystal graph neural networks trained on large datasets of relaxed to predict the relaxed formation energies of unrelaxed structures [1]. This augmentation improves predictions for unrelaxed structures by 66%. Second, we combine effective many-body potentials in a cubic B-spline basis with regularized linear regression to obtain machine-learning potentials that are physically interpretable, sufficiently accurate for applications, and as fast as the fastest traditional empirical potentials [2]. We show that these ultra-fast potentials are two to four orders of magnitude faster than state-of-the-art machine-learning potentials but close in accuracy and enable rapid relaxations and dynamics simulations of large atomistic systems. Finally, we show that symbolic regression methods coupled with physical insights can discover empirical relations and improve previous equations, such as the Allen-Dynes equation for predictions of superconducting transition temperatures based on the electron-phonon spectral function [3,4]. These three methods demonstrate the power of machine learning to accelerate the discovery and design of materials and illustrate some of the issues for developing efficient complex workflows for materials.
[1] Data-augmentation for graph neural network learning of the relaxed energies of unrelaxed structures.
J. Gibson, A. C. Hire, and R. G. Hennig, npj Computational Materials 8, 2115 (2022), doi:10.1038/s41524-022-00891-8.
[2] Ultra-fast interpretable machine-learning potentials. S. R. Xie, M. Rupp, and R. G. Hennig, arXiv:2110.00624 (2020), doi:10.48550/arXiv.2110.00624.
[3] Machine learning of superconducting critical temperature from Eliashberg theory. S. R. Xie, Y. Quan, A. C. Hire, B. Deng, J. M. DeStefano, I. Salinas, U. S. Shah, L. Fanfarillo, J. Lim, J. Kim, G. R. Stewart, J. J. Hamlin, P. J. Hirschfeld, and R. G. Hennig, npj Computational Materials 8, 1 (2022), doi:10.1038/s41524-021-00666-7.
[4] Functional Form of the Superconducting Critical Temperature from Machine Learning. S. R. Xie, G. R. Stewart, J. J. Hamlin, P. J. Hirschfeld, and R. G. Hennig, Phys. Rev. B 100, 174513 (2019), doi:10.1103/PhysRevB.100.174513.
Learn more online at: hhttp://www.ipam.ucla.edu/programs/workshops/workshop-iii-complex-scientific-workflows-at-extreme-computational-scales/
Видео Richard Hennig & Jason Gibson - AI-driven workflows for the discovery of novel superconductors канала Institute for Pure & Applied Mathematics (IPAM)
Показать
Комментарии отсутствуют
Информация о видео
6 мая 2023 г. 1:12:05
00:52:47
Другие видео канала
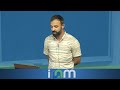
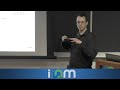
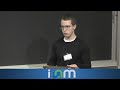
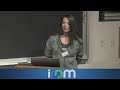
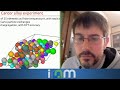
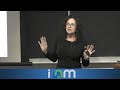
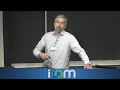
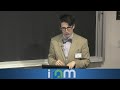
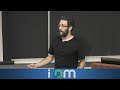
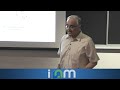
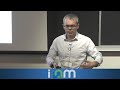
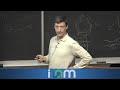
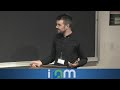
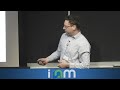
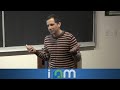
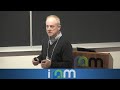
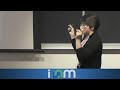
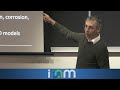
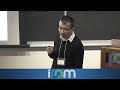
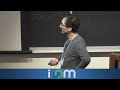