Frederic Legoll - Parareal algorithms for molecular dynamics simulations - IPAM at UCLA
Recorded 21 April 2023. Frederic Legoll of Ecole Nationale Des Ponts et Chaussees presents "Parareal algorithms for molecular dynamics simulations" at IPAM's workshop for Scale-Bridging Materials Modeling at Extreme Computational Scales.
Abstract: In this talk, we consider parareal algorithms in the context of molecular dynamics simulations. The parareal algorithm, which has been originally proposed two decades ago, efficiently solves evolution problems by using parallel-in-time computations. It is based on a decomposition of the time interval into subintervals and makes use of a predictor-corrector strategy. It first uses a coarse, inexpensive solver to quickly step through the whole time domain, and next refines this approximate solution by using an accurate fine solver which is applied concurrently, in parallel, over each time subinterval (assuming that many processors are available). The prediction-correction process is repeated until convergence is reached.
Although the parareal algorithm, in its original formulation, always converges, it suffers from various limitations in the context of molecular dynamics. In particular, it is observed that the algorithm does not provide any computational gain (in terms of wall-clock time compared to a standard sequential integration) in the limit of increasingly long time-horizons. This numerical observation is backed up with theoretical discussions. We introduce here a modified version of the parareal algorithm where the algorithm adaptively divides the entire time-horizon into smaller time slabs. We numerically show that the adaptive algorithm overcomes the various limitations of the standard parareal algorithm, thereby allowing for significantly improved gains. Several numerical examples (based on the Langevin equation) will be discussed, including the simulation using the LAMMPS software of defects diffusing in a tungsten lattice.
This talk is based on joint works with O. Gorynina, T. Lelievre, D. Perez and U. Sharma.
Learn more online at: http://www.ipam.ucla.edu/programs/workshops/workshop-ii-scale-bridging-materials-modeling-at-extreme-computational-scales/
Видео Frederic Legoll - Parareal algorithms for molecular dynamics simulations - IPAM at UCLA канала Institute for Pure & Applied Mathematics (IPAM)
Abstract: In this talk, we consider parareal algorithms in the context of molecular dynamics simulations. The parareal algorithm, which has been originally proposed two decades ago, efficiently solves evolution problems by using parallel-in-time computations. It is based on a decomposition of the time interval into subintervals and makes use of a predictor-corrector strategy. It first uses a coarse, inexpensive solver to quickly step through the whole time domain, and next refines this approximate solution by using an accurate fine solver which is applied concurrently, in parallel, over each time subinterval (assuming that many processors are available). The prediction-correction process is repeated until convergence is reached.
Although the parareal algorithm, in its original formulation, always converges, it suffers from various limitations in the context of molecular dynamics. In particular, it is observed that the algorithm does not provide any computational gain (in terms of wall-clock time compared to a standard sequential integration) in the limit of increasingly long time-horizons. This numerical observation is backed up with theoretical discussions. We introduce here a modified version of the parareal algorithm where the algorithm adaptively divides the entire time-horizon into smaller time slabs. We numerically show that the adaptive algorithm overcomes the various limitations of the standard parareal algorithm, thereby allowing for significantly improved gains. Several numerical examples (based on the Langevin equation) will be discussed, including the simulation using the LAMMPS software of defects diffusing in a tungsten lattice.
This talk is based on joint works with O. Gorynina, T. Lelievre, D. Perez and U. Sharma.
Learn more online at: http://www.ipam.ucla.edu/programs/workshops/workshop-ii-scale-bridging-materials-modeling-at-extreme-computational-scales/
Видео Frederic Legoll - Parareal algorithms for molecular dynamics simulations - IPAM at UCLA канала Institute for Pure & Applied Mathematics (IPAM)
Показать
Комментарии отсутствуют
Информация о видео
22 апреля 2023 г. 2:43:54
00:45:05
Другие видео канала
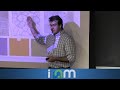
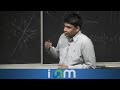
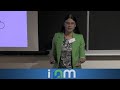
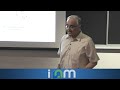
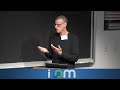
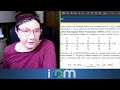
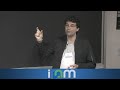
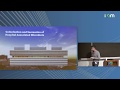
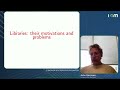
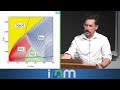
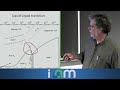
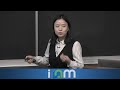
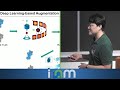
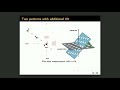
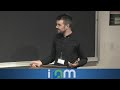
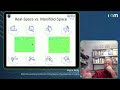
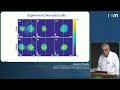
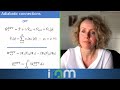
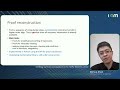
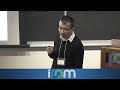
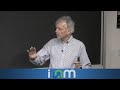