Michele Ceriotti - Machine learning for atomic-scale modeling - potentials and beyond - IPAM at UCLA
Recorded 03 May 2023. Michele Ceriotti of the École Polytechnique Fédérale de Lausanne (EPFL) presents "Machine learning for atomic-scale modeling - potentials and beyond" at IPAM's workshop for Complex Scientific Workflows at Extreme Computational Scales.
Abstract: Over the past decade, interatomic potentials based on machine learning (ML) techniques have become an indispensable tool in the atomic-scale modeling of materials. Trained on energies and forces obtained from electronic-structure calculations, they inherit their predictive accuracy, and greatly extend the length and time scales that are accessible to explicit atomistic simulations. In this talk I will focus on two outstanding challenges for the field, limiting an even more widespread adoption.
First, I will discuss the poor scaling of the most widespread models with the degree of chemical diversity - both in terms of the computational cost, and in terms of the amount of training data needed to achieve an accuracy comparable to first-principles calculations.
I will discuss the use of a scheme that compresses chemical information in a lower-dimensional space, reducing dramatically the cost of the model with negligible loss of accuracy, and its application to the construction of a potential that can describe 25 \emph{d}-block transition metals, and describe its use in the modeling of high-entropy alloys.
I will then tackle a more far-reaching issue: electronic-structure calculations give access to functional properties beyond interatomic potentials, and these should also be incorporated into machine-learning frameworks. I will discuss how to achieve a seamless connection between quantum and data driven approaches, and present several examples of simulations in which these two paradigms converge: from models of the electronic charge density and the single-particle
Hamiltonian, to the use of the electron density of states to perform simulations of matter with thermally-excited electrons.
Learn more online at: hhttp://www.ipam.ucla.edu/programs/workshops/workshop-iii-complex-scientific-workflows-at-extreme-computational-scales/
Видео Michele Ceriotti - Machine learning for atomic-scale modeling - potentials and beyond - IPAM at UCLA канала Institute for Pure & Applied Mathematics (IPAM)
Abstract: Over the past decade, interatomic potentials based on machine learning (ML) techniques have become an indispensable tool in the atomic-scale modeling of materials. Trained on energies and forces obtained from electronic-structure calculations, they inherit their predictive accuracy, and greatly extend the length and time scales that are accessible to explicit atomistic simulations. In this talk I will focus on two outstanding challenges for the field, limiting an even more widespread adoption.
First, I will discuss the poor scaling of the most widespread models with the degree of chemical diversity - both in terms of the computational cost, and in terms of the amount of training data needed to achieve an accuracy comparable to first-principles calculations.
I will discuss the use of a scheme that compresses chemical information in a lower-dimensional space, reducing dramatically the cost of the model with negligible loss of accuracy, and its application to the construction of a potential that can describe 25 \emph{d}-block transition metals, and describe its use in the modeling of high-entropy alloys.
I will then tackle a more far-reaching issue: electronic-structure calculations give access to functional properties beyond interatomic potentials, and these should also be incorporated into machine-learning frameworks. I will discuss how to achieve a seamless connection between quantum and data driven approaches, and present several examples of simulations in which these two paradigms converge: from models of the electronic charge density and the single-particle
Hamiltonian, to the use of the electron density of states to perform simulations of matter with thermally-excited electrons.
Learn more online at: hhttp://www.ipam.ucla.edu/programs/workshops/workshop-iii-complex-scientific-workflows-at-extreme-computational-scales/
Видео Michele Ceriotti - Machine learning for atomic-scale modeling - potentials and beyond - IPAM at UCLA канала Institute for Pure & Applied Mathematics (IPAM)
Показать
Комментарии отсутствуют
Информация о видео
4 мая 2023 г. 3:52:17
00:50:39
Другие видео канала
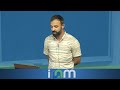
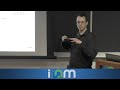
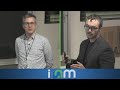
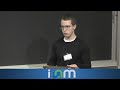
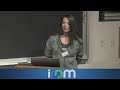
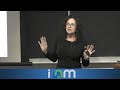
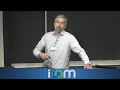
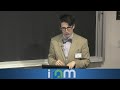
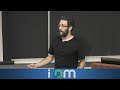
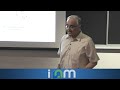
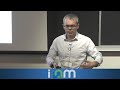
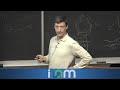
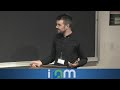
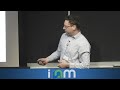
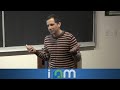
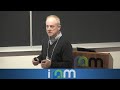
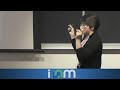
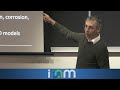
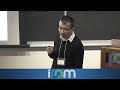
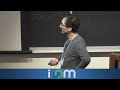