Justin Smith - The state of neural network interatomic potentials - IPAM at UCLA
Recorded 22 May 2023. Justin Smith of NVIDIA presents "The state of neural network interatomic potentials" at IPAM's workshop for Co-design for the Exascale and IPAM Hackathon.
Abstract: Atomistic simulation is a tool of immense power for research in biochemistry, material science and reactive chemistry. There are two traditional approaches to calculating the atomic forces that drive these simulations: classical potentials and ab initio quantum chemistry. Classical potentials are often favored for their high numerical efficiency but lack the flexibility to be accurate across a large application space. Another option is to obtain forces through ab initio quantum chemistry calculations, which tend to be more accurate, but are also more computationally complex. Neural network interatomic potentials (NNIP) have made significant progress towards bridging this speed vs. accuracy gap over the last decade. Regardless of this progress, in many domains of research there are still significant challenges preventing widespread adoption and application of NNIPs. Challenges such as ideal training data set generation, model validation, bespoke vs. general models, computational speed compared to classical approaches, and lack of out-of-the-box workflows and trained models for non-NNIP experts to deploy in their research. In this presentation I will discuss NNIPs, their strengths and weaknesses vs. other approaches, the blockers to adoption for various application domains, how these blockers relate across domains, and various ongoing research and software engineering efforts to find solutions.
Learn more online at: http://www.ipam.ucla.edu/programs/workshops/workshop-iv-co-design-for-the-exascale-and-ipam-hackathon/?tab=overview
Видео Justin Smith - The state of neural network interatomic potentials - IPAM at UCLA канала Institute for Pure & Applied Mathematics (IPAM)
Abstract: Atomistic simulation is a tool of immense power for research in biochemistry, material science and reactive chemistry. There are two traditional approaches to calculating the atomic forces that drive these simulations: classical potentials and ab initio quantum chemistry. Classical potentials are often favored for their high numerical efficiency but lack the flexibility to be accurate across a large application space. Another option is to obtain forces through ab initio quantum chemistry calculations, which tend to be more accurate, but are also more computationally complex. Neural network interatomic potentials (NNIP) have made significant progress towards bridging this speed vs. accuracy gap over the last decade. Regardless of this progress, in many domains of research there are still significant challenges preventing widespread adoption and application of NNIPs. Challenges such as ideal training data set generation, model validation, bespoke vs. general models, computational speed compared to classical approaches, and lack of out-of-the-box workflows and trained models for non-NNIP experts to deploy in their research. In this presentation I will discuss NNIPs, their strengths and weaknesses vs. other approaches, the blockers to adoption for various application domains, how these blockers relate across domains, and various ongoing research and software engineering efforts to find solutions.
Learn more online at: http://www.ipam.ucla.edu/programs/workshops/workshop-iv-co-design-for-the-exascale-and-ipam-hackathon/?tab=overview
Видео Justin Smith - The state of neural network interatomic potentials - IPAM at UCLA канала Institute for Pure & Applied Mathematics (IPAM)
Показать
Комментарии отсутствуют
Информация о видео
23 мая 2023 г. 0:51:53
00:41:23
Другие видео канала
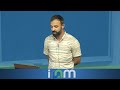
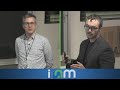
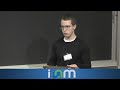
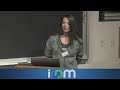
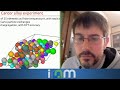
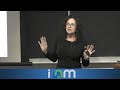
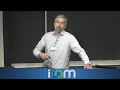
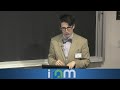
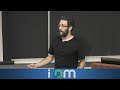
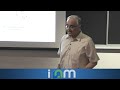
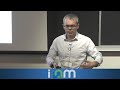
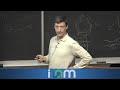
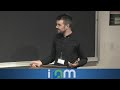
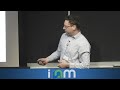
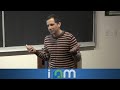
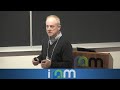
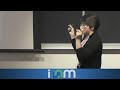
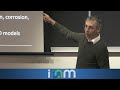
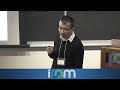
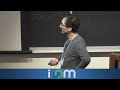