Christoph Ortner - Atomic Cluster Expansion with and Without Atoms - IPAM at UCLA
Recorded 19 April 2023. Christoph Ortner of the University of British Columbia presents "Atomic Cluster Expansion with and Without Atoms" at IPAM's workshop for Scale-Bridging Materials Modeling at Extreme Computational Scales.
Abstract: I will outline a geometric learning framework, the atomic cluster expansion (ACE), which focuses on linear and shallow models. The framework is particularly well-suited for studying particle systems where it often achieves state-of-the-art accuracy / computational performance and such it is well suited for applications requiring high performance. I will first review the general setting, i.e., how the ACE concept can be adapted to provide models in many different contexts, including molecular simulation, electronic structure, wave functions, and particle physics.
In the second part of my talk I will then review an emerging framework or workflow - hyper-active learning - how to efficiently generate training data for ACE models in an automated way. Relatively small and linear ACE models are ideally suited to this setting. I will also go into some details of the priors we impose that make the workflow as robust as possible.
Learn more online at: http://www.ipam.ucla.edu/programs/workshops/workshop-ii-scale-bridging-materials-modeling-at-extreme-computational-scales/
Видео Christoph Ortner - Atomic Cluster Expansion with and Without Atoms - IPAM at UCLA канала Institute for Pure & Applied Mathematics (IPAM)
Abstract: I will outline a geometric learning framework, the atomic cluster expansion (ACE), which focuses on linear and shallow models. The framework is particularly well-suited for studying particle systems where it often achieves state-of-the-art accuracy / computational performance and such it is well suited for applications requiring high performance. I will first review the general setting, i.e., how the ACE concept can be adapted to provide models in many different contexts, including molecular simulation, electronic structure, wave functions, and particle physics.
In the second part of my talk I will then review an emerging framework or workflow - hyper-active learning - how to efficiently generate training data for ACE models in an automated way. Relatively small and linear ACE models are ideally suited to this setting. I will also go into some details of the priors we impose that make the workflow as robust as possible.
Learn more online at: http://www.ipam.ucla.edu/programs/workshops/workshop-ii-scale-bridging-materials-modeling-at-extreme-computational-scales/
Видео Christoph Ortner - Atomic Cluster Expansion with and Without Atoms - IPAM at UCLA канала Institute for Pure & Applied Mathematics (IPAM)
Показать
Комментарии отсутствуют
Информация о видео
20 апреля 2023 г. 1:35:49
00:53:04
Другие видео канала
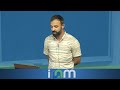
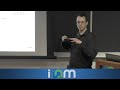
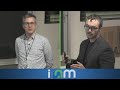
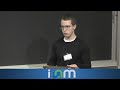
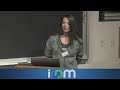
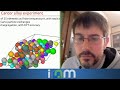
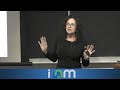
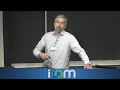
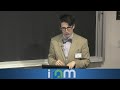
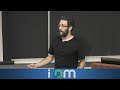
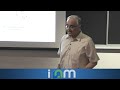
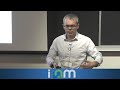
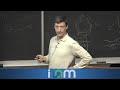
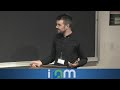
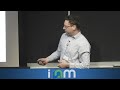
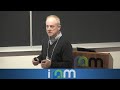
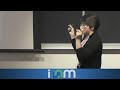
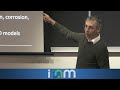
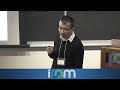
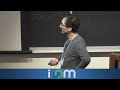