Tatiana Bubba - Deep neural networks for inverse problems with pseudodifferential operators
Presentation given by Tatiana Bubba on 4 August 2021 in the one world seminar on the mathematics of machine learning on the topic "Deep neural networks for inverse problems with pseudodifferential operators: an application to limited-angle tomography".
Abstract: In this talk, I present a novel convolutional neural network (CNN), called $\Psi$DONet, designed for learning pseudodifferential operators in the context of linear inverse problems. The starting point is the Iterative Soft Thresholding Algorithm (ISTA), a well-known algorithm to solve sparsity-promoting minimization problems. I will show that, under rather general assumptions on the forward operator, the unfolded iterations of ISTA can be interpreted as the successive layers of a CNN. In turn this provides fairly general network architectures that, for a specific choice of the parameters involved, allow to reproduce ISTA, or a perturbation of ISTA for which the coefficients of the filters can be bounded. I will use as case study the limited-angle X-ray transform and its application to limited-angle computed tomography (LA-CT). In particular, I will prove that, in the case of LA-CT, the operations of upscaling, downscaling and convolution, which characterize $\Psi$DONet and most deep learning schemes, can be exactly determined by combining the convolutional nature of the limited angle X-ray transform and basic properties defining an orthogonal wavelet system. Finally, I propose two different implementations of $\Psi$DONet which are tested on simulated data from LA geometry, generated from the ellipse data set. This is a joint work with L. Ratti, M. Galinier, M. Lassas, M. Prato and S. Siltanen.
Видео Tatiana Bubba - Deep neural networks for inverse problems with pseudodifferential operators канала One world theoretical machine learning
Abstract: In this talk, I present a novel convolutional neural network (CNN), called $\Psi$DONet, designed for learning pseudodifferential operators in the context of linear inverse problems. The starting point is the Iterative Soft Thresholding Algorithm (ISTA), a well-known algorithm to solve sparsity-promoting minimization problems. I will show that, under rather general assumptions on the forward operator, the unfolded iterations of ISTA can be interpreted as the successive layers of a CNN. In turn this provides fairly general network architectures that, for a specific choice of the parameters involved, allow to reproduce ISTA, or a perturbation of ISTA for which the coefficients of the filters can be bounded. I will use as case study the limited-angle X-ray transform and its application to limited-angle computed tomography (LA-CT). In particular, I will prove that, in the case of LA-CT, the operations of upscaling, downscaling and convolution, which characterize $\Psi$DONet and most deep learning schemes, can be exactly determined by combining the convolutional nature of the limited angle X-ray transform and basic properties defining an orthogonal wavelet system. Finally, I propose two different implementations of $\Psi$DONet which are tested on simulated data from LA geometry, generated from the ellipse data set. This is a joint work with L. Ratti, M. Galinier, M. Lassas, M. Prato and S. Siltanen.
Видео Tatiana Bubba - Deep neural networks for inverse problems with pseudodifferential operators канала One world theoretical machine learning
Показать
Комментарии отсутствуют
Информация о видео
17 августа 2021 г. 15:42:25
00:45:40
Другие видео канала
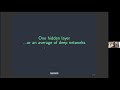
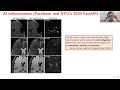
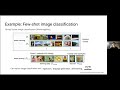
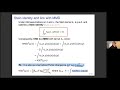
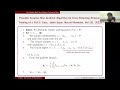
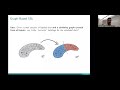
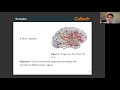
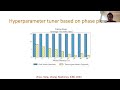
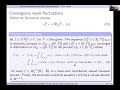
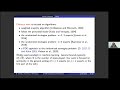
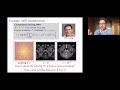
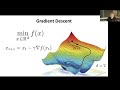
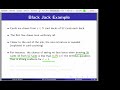
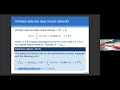
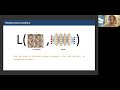
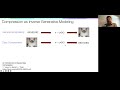

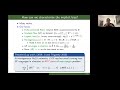
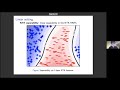
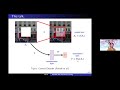
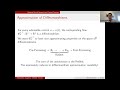