Anirbit Mukherjee - Provable Training of Neural Nets With One Layer of Activation
Abstract: Provable neural training is a fundamental challenge in the field of deep-learning theory – and it largely remains an open question for almost any neural net of practical relevance. The quest for provable convergence for neural training algorithms almost always leads to exciting new questions in mathematics. In this talk, I shall give an overview of three convergence proofs of ours in this territory: (1) In 2016 we had shown the first deterministic algorithm that converges to the exact global minima of any convex loss function for any depth 2 ReLU neural net for any training data in time that is only polynomial in the training data size. (2) In 2020 we showed the first stochastic algorithm that converges to the global minima of a single ReLU gate in linear time (exponentially fast convergence) for realizable data whilst not assuming any specific distribution for the inputs. (3) In 2022, in a first-of-its-kind result we leveraged the theory of SDEs and Villani functions to show that SGD converges to the global minima of an appropriately Frobenius norm regularized squared loss on any depth 2 neural net with tanh or sigmoid activations – for arbitrary width and data. We shall end the talk delineating various open questions in this direction that can possibly be tackled in the near future.
Видео Anirbit Mukherjee - Provable Training of Neural Nets With One Layer of Activation канала One world theoretical machine learning
Видео Anirbit Mukherjee - Provable Training of Neural Nets With One Layer of Activation канала One world theoretical machine learning
Показать
Комментарии отсутствуют
Информация о видео
9 марта 2023 г. 23:58:14
00:43:24
Другие видео канала
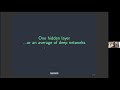
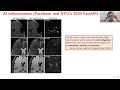
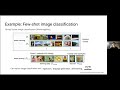
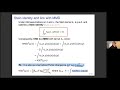
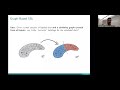
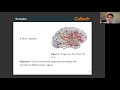
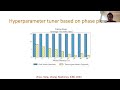
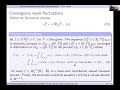
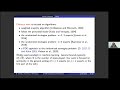
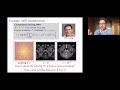
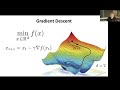
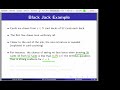
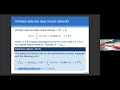
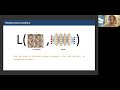
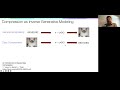

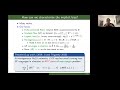
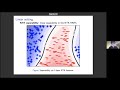
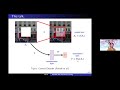
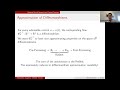