Marcus Hutter - Testing Independence of Exchangeable Random Variables
Abstract: Given well-shuffled data, can we determine whether the data items are statistically (in)dependent? Formally, we consider the problem of testing whether a set of exchangeable random variables are independent. We will show that this is possible and develop tests that can confidently reject the null hypothesis that data is independent and identically distributed and have high power for (some) exchangeable distributions. We will make no structural assumptions on the underlying sample space. One potential application is in Deep Learning, where data is often scraped from the whole internet, with duplications abound, which can render data non-iid and test-set evaluation prone to give wrong answers.
Видео Marcus Hutter - Testing Independence of Exchangeable Random Variables канала One world theoretical machine learning
Видео Marcus Hutter - Testing Independence of Exchangeable Random Variables канала One world theoretical machine learning
Показать
Комментарии отсутствуют
Информация о видео
7 ноября 2022 г. 10:33:00
00:58:22
Другие видео канала
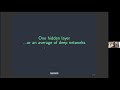
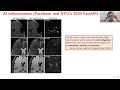
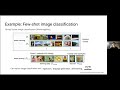
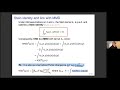
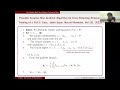
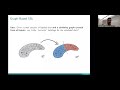
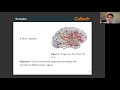
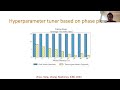
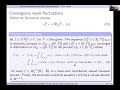
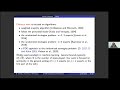
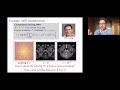
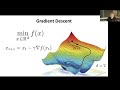
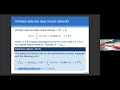
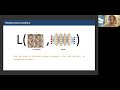
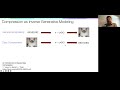

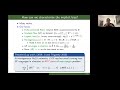
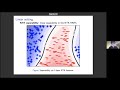
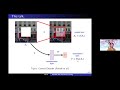
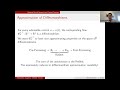