EI Seminar - Grey Yang - Tuning GPT-3 on a Single GPU via Zero-Shot Hyperparameter Transfer
ABSTRACT: You can’t train GPT-3 on a single GPU, much less tune its hyperparameters (HPs)…or so it seems. I’m here to tell you this is not true: you *can* tune its HPs on a single GPU even if you can’t train it that way! In the first half of this talk, I’ll describe how, in the so-called maximal update parametrization (abbreviated µP), narrow and wide neural networks share the same set of optimal HPs. This lets us tune any large model by just tuning a small version of it — we call this µTransfer. In particular, this allowed us to tune the 6.7 billion parameter version of GPT-3 using only 7% of its pretraining compute budget, and, with some asterisks, we get a performance comparable to the original GPT-3 model with twice the parameter count. In the second half of this talk, I’ll discuss the theoretical reason µP has this special property and the connection to the study of infinite-width neural networks and, more generally, the theory of Tensor Programs. The first half will target general practitioners or empirical researchers in machine learning, while the second half targets those who are more theoretically curious. This talk is based on http://arxiv.org/abs/2203.03466.
BIO: Greg Yang is a researcher at Microsoft Research in Redmond, Washington. He joined MSR after he obtained Bachelor’s in Mathematics and Master’s degrees in Computer Science from Harvard University, respectively advised by ST Yau and Alexander Rush. He won the Hoopes prize at Harvard for best undergraduate thesis as well as Honorable Mention for the AMS-MAA-SIAM Morgan Prize, the highest honor in the world for an undergraduate in mathematics. He gave an invited talk at the International Congress of Chinese Mathematicians 2019.
Видео EI Seminar - Grey Yang - Tuning GPT-3 on a Single GPU via Zero-Shot Hyperparameter Transfer канала MIT Embodied Intelligence
BIO: Greg Yang is a researcher at Microsoft Research in Redmond, Washington. He joined MSR after he obtained Bachelor’s in Mathematics and Master’s degrees in Computer Science from Harvard University, respectively advised by ST Yau and Alexander Rush. He won the Hoopes prize at Harvard for best undergraduate thesis as well as Honorable Mention for the AMS-MAA-SIAM Morgan Prize, the highest honor in the world for an undergraduate in mathematics. He gave an invited talk at the International Congress of Chinese Mathematicians 2019.
Видео EI Seminar - Grey Yang - Tuning GPT-3 on a Single GPU via Zero-Shot Hyperparameter Transfer канала MIT Embodied Intelligence
Показать
Комментарии отсутствуют
Информация о видео
Другие видео канала
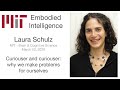
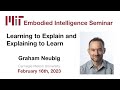

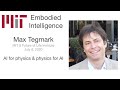
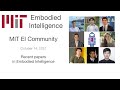
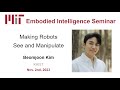
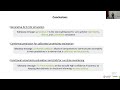
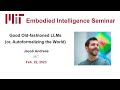
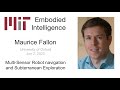
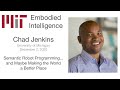
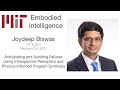
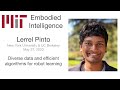
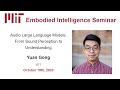
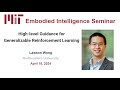
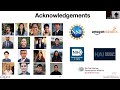
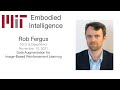
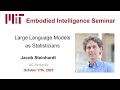
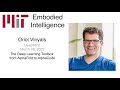
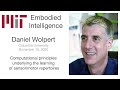
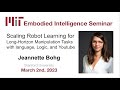