EI Seminar - Joydeep Biswas
Title:
Anticipating and Avoiding Failures Using Introspective Perception and Physics-Informed Program Synthesis
Abstract:
Robots deployed over extended periods of time in unstructured human environments will inevitably make mistakes in perception and planning due to violations of algorithmic assumptions, unmodelled phenomena, and environment-specific constraints. In this talk, I will present our recent work on two fronts to anticipate and overcome such errors: introspective perception to learn to predict and overcome perception errors, and physics-informed program synthesis of action selection policies to enable environment- and user-specific customizability. Introspective perception leverages either an occasionally available supervisory sensor or post-hoc analysis to autonomously train competency-prediction models of black-box perception algorithms. We present results of introspective perception applied to vision-based obstacle avoidance, showing accurate predictions of algorithm-specific errors. We further use introspective perception to learn context-specific noise models for simultaneous localization and mapping, resulting in significant improvements to accuracy and robustness in visually challenging real-world settings.
To adapt the behavior of robots based on user preferences, or to handle unforeseen circumstances, we synthesize and repair programmatic action selection policies (ASPs) in a human-interpretable domain-specific language (DSL), leveraging three key insights: 1) robot ASPs can be represented in a layered structure where each layer consists of increasingly complex subsets of the DSL grammar; 2) physics-informed constraints can be expressed in a type system to prune the search space of programs; and 3) using partial evaluation and human corrections, parameters of such ASPs can be repaired by expressing the corrections to the ASP as satisfiability modulo theory (SMT) formulas. We demonstrate how physics-informed program synthesis can generate ASPs that rival expert-written reference ASPs, require orders of magnitude fewer demonstrations to synthesize than using deep learning, and are repairable when transferred between domains, such as from simulation to real robots.
Bio:
Joydeep Biswas is an assistant professor in the department of computer science at the University of Texas at Austin. He earned his B.Tech in Engineering Physics from the Indian Institute of Technology Bombay in 2008, and M.S. and PhD in Robotics from Carnegie Mellon University in 2010 and 2014 respectively. From 2015 to 2019, he was assistant professor in the College of Information and Computer Sciences at the University of Massachusetts Amherst. His research spans perception and planning for long-term autonomy, with the ultimate goal of having service mobile robots deployed in human environments for years at a time, without the need for expert corrections or supervision. Prof. Biswas' research is supported by funding from NSF, DARPA, Army Futures Command Robotics Center of Excellence, an Amazon Research Award, a JP Morgan Faculty Research Award, and by Northrop Grumman Mission Systems.
Видео EI Seminar - Joydeep Biswas канала MIT Embodied Intelligence
Anticipating and Avoiding Failures Using Introspective Perception and Physics-Informed Program Synthesis
Abstract:
Robots deployed over extended periods of time in unstructured human environments will inevitably make mistakes in perception and planning due to violations of algorithmic assumptions, unmodelled phenomena, and environment-specific constraints. In this talk, I will present our recent work on two fronts to anticipate and overcome such errors: introspective perception to learn to predict and overcome perception errors, and physics-informed program synthesis of action selection policies to enable environment- and user-specific customizability. Introspective perception leverages either an occasionally available supervisory sensor or post-hoc analysis to autonomously train competency-prediction models of black-box perception algorithms. We present results of introspective perception applied to vision-based obstacle avoidance, showing accurate predictions of algorithm-specific errors. We further use introspective perception to learn context-specific noise models for simultaneous localization and mapping, resulting in significant improvements to accuracy and robustness in visually challenging real-world settings.
To adapt the behavior of robots based on user preferences, or to handle unforeseen circumstances, we synthesize and repair programmatic action selection policies (ASPs) in a human-interpretable domain-specific language (DSL), leveraging three key insights: 1) robot ASPs can be represented in a layered structure where each layer consists of increasingly complex subsets of the DSL grammar; 2) physics-informed constraints can be expressed in a type system to prune the search space of programs; and 3) using partial evaluation and human corrections, parameters of such ASPs can be repaired by expressing the corrections to the ASP as satisfiability modulo theory (SMT) formulas. We demonstrate how physics-informed program synthesis can generate ASPs that rival expert-written reference ASPs, require orders of magnitude fewer demonstrations to synthesize than using deep learning, and are repairable when transferred between domains, such as from simulation to real robots.
Bio:
Joydeep Biswas is an assistant professor in the department of computer science at the University of Texas at Austin. He earned his B.Tech in Engineering Physics from the Indian Institute of Technology Bombay in 2008, and M.S. and PhD in Robotics from Carnegie Mellon University in 2010 and 2014 respectively. From 2015 to 2019, he was assistant professor in the College of Information and Computer Sciences at the University of Massachusetts Amherst. His research spans perception and planning for long-term autonomy, with the ultimate goal of having service mobile robots deployed in human environments for years at a time, without the need for expert corrections or supervision. Prof. Biswas' research is supported by funding from NSF, DARPA, Army Futures Command Robotics Center of Excellence, an Amazon Research Award, a JP Morgan Faculty Research Award, and by Northrop Grumman Mission Systems.
Видео EI Seminar - Joydeep Biswas канала MIT Embodied Intelligence
Показать
Комментарии отсутствуют
Информация о видео
Другие видео канала
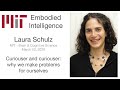
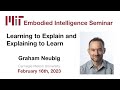

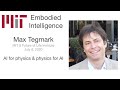
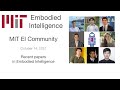
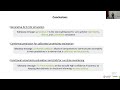
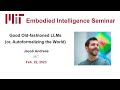
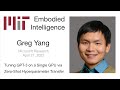
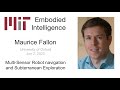
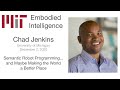
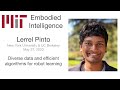
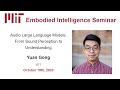
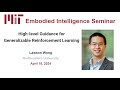
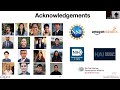
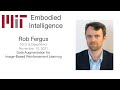
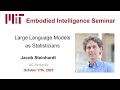
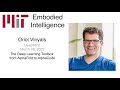
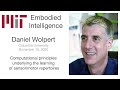
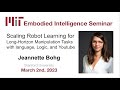
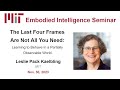