Jane Dwivedi Yu - Teaching Language models to use tools | ML in PL 23
Large language models (LLMs) have fueled dramatic progress in natural language tasks and are already at the core of many user-facing products, such as ChatGPT and Copilot. Paradoxically, language models often still struggle with basic tasks, like solving simple arithmetic problems, where smaller and simpler external resources, such as a calculator, can accomplish the task perfectly. This talk will focus on LLMs that leverage external resources, beginning with models that are always prompted to use an external tool, like retrieval-augmented models. The second part of this talk will concentrate on teaching models to autonomously understand how and when to leverage tools in a self-supervised way. Finally, we will discuss exciting new opportunities that necessitate external tool usage.
Jane Dwivedi-Yu is a researcher at Meta AI. Her current research focuses on enhancing capabilities of language models along several dimensions, including tool usage, editing, and evaluating representation harms and notions of morality and norms internalized by these models. She is also interested in building large-scale personalized recommender systems by leveraging principles from affective computing, work which was cited among the top 15 AI papers to read in 2022. Before joining Meta, she completed her PhD in Computer Science at University of California, Berkeley and Bachelors at Cornell University.
The talk was delivered during ML in PL Conference 2023 as a part of Contributed Talks. The conference was organized by a non-profit NGO called ML in PL Association.
ML in PL Association website: https://mlinpl.org/
ML In PL Conference 2023 website: https://conference2023.mlinpl.org/
ML In PL Conference 2024 website: https://conference.mlinpl.org/
---
ML in PL Association was founded based on the experiences in organizing of the ML in PL Conference (formerly PL in ML), the ML in PL Association is a non-profit organization devoted to fostering the machine learning community in Poland and Europe and promoting a deep understanding of ML methods. Even though ML in PL is based in Poland, it seeks to provide opportunities for international cooperation.
Видео Jane Dwivedi Yu - Teaching Language models to use tools | ML in PL 23 канала ML in PL
Jane Dwivedi-Yu is a researcher at Meta AI. Her current research focuses on enhancing capabilities of language models along several dimensions, including tool usage, editing, and evaluating representation harms and notions of morality and norms internalized by these models. She is also interested in building large-scale personalized recommender systems by leveraging principles from affective computing, work which was cited among the top 15 AI papers to read in 2022. Before joining Meta, she completed her PhD in Computer Science at University of California, Berkeley and Bachelors at Cornell University.
The talk was delivered during ML in PL Conference 2023 as a part of Contributed Talks. The conference was organized by a non-profit NGO called ML in PL Association.
ML in PL Association website: https://mlinpl.org/
ML In PL Conference 2023 website: https://conference2023.mlinpl.org/
ML In PL Conference 2024 website: https://conference.mlinpl.org/
---
ML in PL Association was founded based on the experiences in organizing of the ML in PL Conference (formerly PL in ML), the ML in PL Association is a non-profit organization devoted to fostering the machine learning community in Poland and Europe and promoting a deep understanding of ML methods. Even though ML in PL is based in Poland, it seeks to provide opportunities for international cooperation.
Видео Jane Dwivedi Yu - Teaching Language models to use tools | ML in PL 23 канала ML in PL
Показать
Комментарии отсутствуют
Информация о видео
Другие видео канала
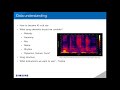
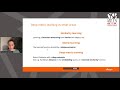
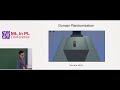
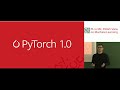

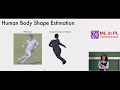
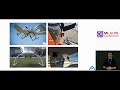
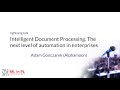
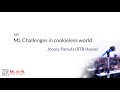
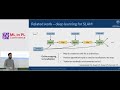


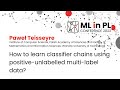
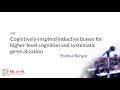

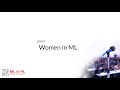
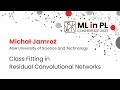
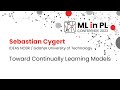
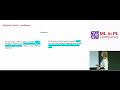
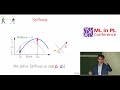
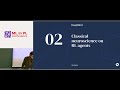