Agnieszka Grabska-Barwińska - Neuroscience-inspired analysis of machine learning
Recorded at the ML in PL 2019 Conference, the University of Warsaw, 22-24 November 2019.
Agnieszka Grabska-Barwińska (Google Deepmind)
Abstract:
Machine learning has recently proven its worth in solving problems that are notoriously difficult for humans, superseding us not just in games such as Chess or Go, but also in real world settings such as control (robots, power plants), recommendation and detection systems. As our machines scale to increasingly ambitious challenges, the importance of understanding their inner workings necessarily increases. Can we understand their decisions? Can we extract the algorithms they implicitly discover? Can we learn from them? Similar such questions have been at the forefront of Neuroscience research for decades. In this talk, I will draw upon many experiences gained during my personal academic journey, which started as a neuroscientist primarily focused on the question of "How does the brain work?" before transitioning into a career primary focused on understanding how artificial minds work. I will present a number of personal case studies dealing with the understanding of modern large-scale artificial intelligence systems, focusing particularly on whether neuroscience-inspired techniques can help us gain insight into the inner workings of artificial minds.
References:
Hubel and Wiesel, 1959
Receptive fields of single neurones in the cat's striate cortex. J Physiol. 1959 Oct; 148: 574-591
https://www.ncbi.nlm.nih.gov/pmc/articles/PMC1363130/
A Grabska‐Barwińska et al., 2009
Contrast independence of cardinal preference: stable oblique effect in orientation maps of ferret visual cortex. European Journal of Neuroscience 29, 1258-1270
http://homepage.ruhr-uni-bochum.de/klaus-peter.hoffmann/pdf_hoffmann/grabska_ejn_09.pdf
Ohki et al., 2005
Functional imaging with cellular resolution reveals precise micro-architecture in visual cortex. Nature 433, 597–603
https://www.nature.com/articles/nature03274
Mnih et al., 2015
Human-level control through deep reinforcement learning. Nature 518(7540), 529–533
https://www.nature.com/articles/nature14236
van Hasselt et al., 2015
Deep Reinforcement Learning with Double Q-learning
https://arxiv.org/abs/1509.06461
Kirkpatrick et al., 2017
Overcoming catastrophic forgetting in neural networks. Proceedings of the national academy of sciences 114, 3521–3526
https://www.pnas.org/content/114/13/3521
J Veness et al., 2017
Online learning with gated linear networks. CoRR
https://arxiv.org/abs/1712.01897
Graves et al., 2016
Hybrid computing using a neural network with dynamic external memory. Nature 538, 471–476
https://www.nature.com/articles/nature20101
Wayne et al., 2018
Unsupervised predictive memory in a goal-directed agent. CoRR
https://arxiv.org/abs/1803.10760
Jaderberg et al., 2019
Human-level performance in 3D multiplayer games with population-based reinforcement learning. Science 364, 859–865
https://science.sciencemag.org/content/364/6443/859
Bapst et al., 2020
Unveiling the predictive power of static structure in glassy systems. Nature Physics 16, 448–454
https://www.nature.com/articles/s41567-020-0842-8
Видео Agnieszka Grabska-Barwińska - Neuroscience-inspired analysis of machine learning канала ML in PL
Agnieszka Grabska-Barwińska (Google Deepmind)
Abstract:
Machine learning has recently proven its worth in solving problems that are notoriously difficult for humans, superseding us not just in games such as Chess or Go, but also in real world settings such as control (robots, power plants), recommendation and detection systems. As our machines scale to increasingly ambitious challenges, the importance of understanding their inner workings necessarily increases. Can we understand their decisions? Can we extract the algorithms they implicitly discover? Can we learn from them? Similar such questions have been at the forefront of Neuroscience research for decades. In this talk, I will draw upon many experiences gained during my personal academic journey, which started as a neuroscientist primarily focused on the question of "How does the brain work?" before transitioning into a career primary focused on understanding how artificial minds work. I will present a number of personal case studies dealing with the understanding of modern large-scale artificial intelligence systems, focusing particularly on whether neuroscience-inspired techniques can help us gain insight into the inner workings of artificial minds.
References:
Hubel and Wiesel, 1959
Receptive fields of single neurones in the cat's striate cortex. J Physiol. 1959 Oct; 148: 574-591
https://www.ncbi.nlm.nih.gov/pmc/articles/PMC1363130/
A Grabska‐Barwińska et al., 2009
Contrast independence of cardinal preference: stable oblique effect in orientation maps of ferret visual cortex. European Journal of Neuroscience 29, 1258-1270
http://homepage.ruhr-uni-bochum.de/klaus-peter.hoffmann/pdf_hoffmann/grabska_ejn_09.pdf
Ohki et al., 2005
Functional imaging with cellular resolution reveals precise micro-architecture in visual cortex. Nature 433, 597–603
https://www.nature.com/articles/nature03274
Mnih et al., 2015
Human-level control through deep reinforcement learning. Nature 518(7540), 529–533
https://www.nature.com/articles/nature14236
van Hasselt et al., 2015
Deep Reinforcement Learning with Double Q-learning
https://arxiv.org/abs/1509.06461
Kirkpatrick et al., 2017
Overcoming catastrophic forgetting in neural networks. Proceedings of the national academy of sciences 114, 3521–3526
https://www.pnas.org/content/114/13/3521
J Veness et al., 2017
Online learning with gated linear networks. CoRR
https://arxiv.org/abs/1712.01897
Graves et al., 2016
Hybrid computing using a neural network with dynamic external memory. Nature 538, 471–476
https://www.nature.com/articles/nature20101
Wayne et al., 2018
Unsupervised predictive memory in a goal-directed agent. CoRR
https://arxiv.org/abs/1803.10760
Jaderberg et al., 2019
Human-level performance in 3D multiplayer games with population-based reinforcement learning. Science 364, 859–865
https://science.sciencemag.org/content/364/6443/859
Bapst et al., 2020
Unveiling the predictive power of static structure in glassy systems. Nature Physics 16, 448–454
https://www.nature.com/articles/s41567-020-0842-8
Видео Agnieszka Grabska-Barwińska - Neuroscience-inspired analysis of machine learning канала ML in PL
Показать
Комментарии отсутствуют
Информация о видео
Другие видео канала
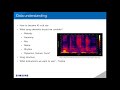
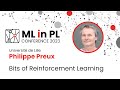
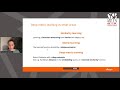
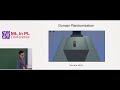
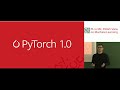

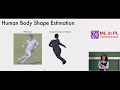
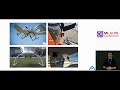
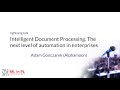
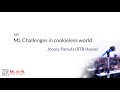
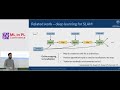


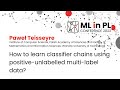
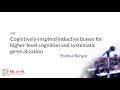

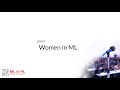
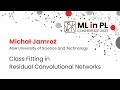
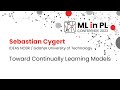
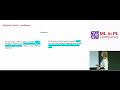
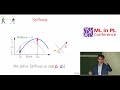