How to learn classifier chains using positive-unlabelled multi-label data? | ML in PL 22
How to learn classifier chains using positive-unlabelled multi-label data? by Paweł Teisseyre (Institute of Computer Science, Polish Academy of Sciences and Faculty of Mathematics and Information Sciences, Warsaw University of Technology)
Multi-label learning deals with data examples which are associated with multiple class labels simultaneously. The problem has attracted significant attention in recent years and dozens of algorithms have been proposed. However in traditional multi-label setting it is assumed that all relevant labels are assigned to the given instance. This assumption is not met in many real-world situations. In positive unlabelled multi-label setting, only some of relevant labels are assigned. The appearance of a label means that the instance is really associated with this label, while the absence of the label does not imply that this label is not proper for the instance. For example, when predicting multiple diseases in one patient, some diseases can be undiagnosed however it does not mean that the patient does not have these diseases. Among many existing multi-label methods, classifier chains gained the great popularity mainly due to their simplicity and high predictive power. However, it turns out that adaptation of classifier chains to positive unlabelled framework is not straightforward, due to the fact that the true target variables are observed only partially and therefore they cannot be used directly to train the models in the chain. The partial observability concerns not only the current target variable in the chain but also the feature space, which additionally increases the difficulty of the problem. We propose two modifications of classifier chains. In the first method we scale the output probabilities of the consecutive classifiers in the chain. In the second method we minimize weighted empirical risk, with weights depending on prior probabilities of the target variables. The predictive performance of the proposed methods is studied on real multi-label datasets for different positive unlabelled settings.
The talk was delivered during ML in PL Conference 2022 as a part of Contributed Talks. The conference was organized by a non-profit NGO called ML in PL Association.
ML in PL Association website: https://mlinpl.org/
ML in PL Conference 2022 website: https://conference2022.mlinpl.org/
ML In PL Conference 2023 website: https://conference2023.mlinpl.org/
---
ML in PL Association was founded based on the experiences in organizing of the ML in PL Conference (formerly PL in ML), the ML in PL Association is a non-profit organization devoted to fostering the machine learning community in Poland and Europe and promoting a deep understanding of ML methods. Even though ML in PL is based in Poland, it seeks to provide opportunities for international cooperation.
Видео How to learn classifier chains using positive-unlabelled multi-label data? | ML in PL 22 канала ML in PL
Multi-label learning deals with data examples which are associated with multiple class labels simultaneously. The problem has attracted significant attention in recent years and dozens of algorithms have been proposed. However in traditional multi-label setting it is assumed that all relevant labels are assigned to the given instance. This assumption is not met in many real-world situations. In positive unlabelled multi-label setting, only some of relevant labels are assigned. The appearance of a label means that the instance is really associated with this label, while the absence of the label does not imply that this label is not proper for the instance. For example, when predicting multiple diseases in one patient, some diseases can be undiagnosed however it does not mean that the patient does not have these diseases. Among many existing multi-label methods, classifier chains gained the great popularity mainly due to their simplicity and high predictive power. However, it turns out that adaptation of classifier chains to positive unlabelled framework is not straightforward, due to the fact that the true target variables are observed only partially and therefore they cannot be used directly to train the models in the chain. The partial observability concerns not only the current target variable in the chain but also the feature space, which additionally increases the difficulty of the problem. We propose two modifications of classifier chains. In the first method we scale the output probabilities of the consecutive classifiers in the chain. In the second method we minimize weighted empirical risk, with weights depending on prior probabilities of the target variables. The predictive performance of the proposed methods is studied on real multi-label datasets for different positive unlabelled settings.
The talk was delivered during ML in PL Conference 2022 as a part of Contributed Talks. The conference was organized by a non-profit NGO called ML in PL Association.
ML in PL Association website: https://mlinpl.org/
ML in PL Conference 2022 website: https://conference2022.mlinpl.org/
ML In PL Conference 2023 website: https://conference2023.mlinpl.org/
---
ML in PL Association was founded based on the experiences in organizing of the ML in PL Conference (formerly PL in ML), the ML in PL Association is a non-profit organization devoted to fostering the machine learning community in Poland and Europe and promoting a deep understanding of ML methods. Even though ML in PL is based in Poland, it seeks to provide opportunities for international cooperation.
Видео How to learn classifier chains using positive-unlabelled multi-label data? | ML in PL 22 канала ML in PL
Показать
Комментарии отсутствуют
Информация о видео
Другие видео канала
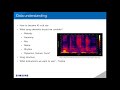
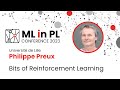
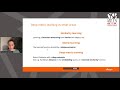
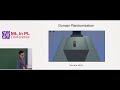
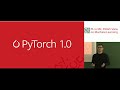

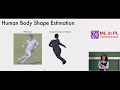
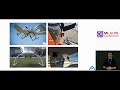
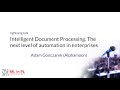
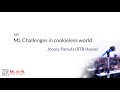
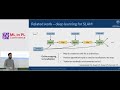


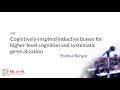

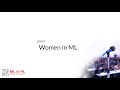
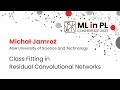
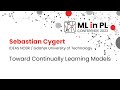
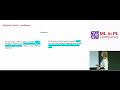
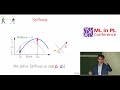