Xavier Bresson: "Graph Convolutional Neural Networks for Molecule Generation"
Machine Learning for Physics and the Physics of Learning 2019
Workshop I: From Passive to Active: Generative and Reinforcement Learning with Physics
"Graph Convolutional Neural Networks for Molecule Generation"
Xavier Bresson, Nanyang Technological University
Abstract: In this talk, I will discuss a graph convolutional neural network architecture for the molecule generation task. The proposed approach consists of two steps. First, a graph ConvNet is used to auto-encode molecules in one-shot. Second, beam search is applied to the output of neural networks to produce a valid chemical solution. Numerical experiments demonstrate the performances of this learning system.
Institute for Pure and Applied Mathematics, UCLA
September 23, 2019
For more information: http://www.ipam.ucla.edu/mlpws1
Видео Xavier Bresson: "Graph Convolutional Neural Networks for Molecule Generation" канала Institute for Pure & Applied Mathematics (IPAM)
Workshop I: From Passive to Active: Generative and Reinforcement Learning with Physics
"Graph Convolutional Neural Networks for Molecule Generation"
Xavier Bresson, Nanyang Technological University
Abstract: In this talk, I will discuss a graph convolutional neural network architecture for the molecule generation task. The proposed approach consists of two steps. First, a graph ConvNet is used to auto-encode molecules in one-shot. Second, beam search is applied to the output of neural networks to produce a valid chemical solution. Numerical experiments demonstrate the performances of this learning system.
Institute for Pure and Applied Mathematics, UCLA
September 23, 2019
For more information: http://www.ipam.ucla.edu/mlpws1
Видео Xavier Bresson: "Graph Convolutional Neural Networks for Molecule Generation" канала Institute for Pure & Applied Mathematics (IPAM)
Показать
Комментарии отсутствуют
Информация о видео
17 декабря 2019 г. 0:55:01
01:00:43
Другие видео канала
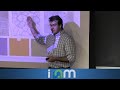
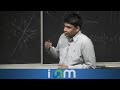
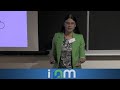
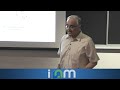
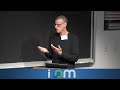
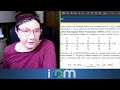
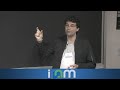
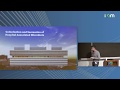
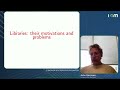
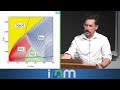
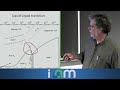
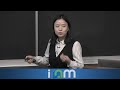
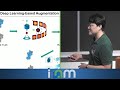
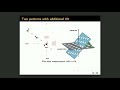
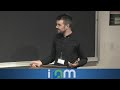
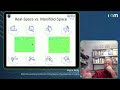
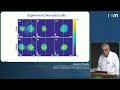
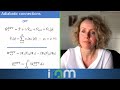
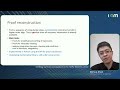
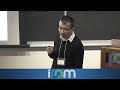
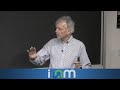