2M All-In into $5 Pot! WWYD? Daniel Negreanu's No-Limit Hold'em Challenge! (Poker Hand Analysis)
#ai #technology #poker
Daniel Negreanu posted a set of very interesting No-Limit Hold'em situations on Twitter. I try to analyze them from the perspective of a poker bot. See how such bots think about the game and approximate Nash equilibria.
https://twitter.com/RealKidPoker/status/1337887509397741568
https://twitter.com/RealKidPoker/status/1337899147337244673
https://twitter.com/RealKidPoker/status/1337904860721606656
Links:
YouTube: https://www.youtube.com/c/yannickilcher
Twitter: https://twitter.com/ykilcher
Discord: https://discord.gg/4H8xxDF
BitChute: https://www.bitchute.com/channel/yannic-kilcher
BiliBili: https://space.bilibili.com/1824646584
Minds: https://www.minds.com/ykilcher
Parler: https://parler.com/profile/YannicKilcher
LinkedIn: https://www.linkedin.com/in/yannic-kilcher-488534136/
If you want to support me, the best thing to do is to share out the content :)
If you want to support me financially (completely optional and voluntary, but a lot of people have asked for this):
SubscribeStar: https://www.subscribestar.com/yannickilcher
Patreon: https://www.patreon.com/yannickilcher
Bitcoin (BTC): bc1q49lsw3q325tr58ygf8sudx2dqfguclvngvy2cq
Ethereum (ETH): 0x7ad3513E3B8f66799f507Aa7874b1B0eBC7F85e2
Litecoin (LTC): LQW2TRyKYetVC8WjFkhpPhtpbDM4Vw7r9m
Monero (XMR): 4ACL8AGrEo5hAir8A9CeVrW8pEauWvnp1WnSDZxW7tziCDLhZAGsgzhRQABDnFy8yuM9fWJDviJPHKRjV4FWt19CJZN9D4n
Видео 2M All-In into $5 Pot! WWYD? Daniel Negreanu's No-Limit Hold'em Challenge! (Poker Hand Analysis) канала Yannic Kilcher
Daniel Negreanu posted a set of very interesting No-Limit Hold'em situations on Twitter. I try to analyze them from the perspective of a poker bot. See how such bots think about the game and approximate Nash equilibria.
https://twitter.com/RealKidPoker/status/1337887509397741568
https://twitter.com/RealKidPoker/status/1337899147337244673
https://twitter.com/RealKidPoker/status/1337904860721606656
Links:
YouTube: https://www.youtube.com/c/yannickilcher
Twitter: https://twitter.com/ykilcher
Discord: https://discord.gg/4H8xxDF
BitChute: https://www.bitchute.com/channel/yannic-kilcher
BiliBili: https://space.bilibili.com/1824646584
Minds: https://www.minds.com/ykilcher
Parler: https://parler.com/profile/YannicKilcher
LinkedIn: https://www.linkedin.com/in/yannic-kilcher-488534136/
If you want to support me, the best thing to do is to share out the content :)
If you want to support me financially (completely optional and voluntary, but a lot of people have asked for this):
SubscribeStar: https://www.subscribestar.com/yannickilcher
Patreon: https://www.patreon.com/yannickilcher
Bitcoin (BTC): bc1q49lsw3q325tr58ygf8sudx2dqfguclvngvy2cq
Ethereum (ETH): 0x7ad3513E3B8f66799f507Aa7874b1B0eBC7F85e2
Litecoin (LTC): LQW2TRyKYetVC8WjFkhpPhtpbDM4Vw7r9m
Monero (XMR): 4ACL8AGrEo5hAir8A9CeVrW8pEauWvnp1WnSDZxW7tziCDLhZAGsgzhRQABDnFy8yuM9fWJDviJPHKRjV4FWt19CJZN9D4n
Видео 2M All-In into $5 Pot! WWYD? Daniel Negreanu's No-Limit Hold'em Challenge! (Poker Hand Analysis) канала Yannic Kilcher
Показать
Комментарии отсутствуют
Информация о видео
Другие видео канала
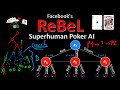
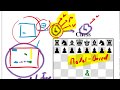
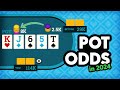
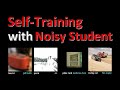
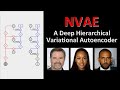
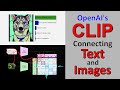
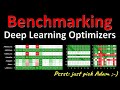
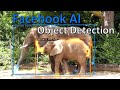
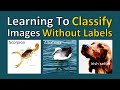
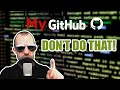
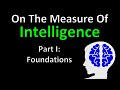
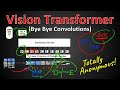
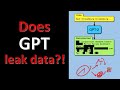
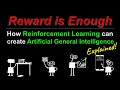
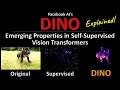
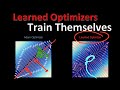
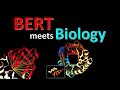
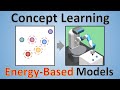
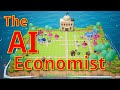
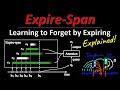