BERTology Meets Biology: Interpreting Attention in Protein Language Models (Paper Explained)
Proteins are the workhorses of almost all cellular functions and a core component of life. But despite their versatility, all proteins are built as sequences of the same 20 amino acids. These sequences can be analyzed with tools from NLP. This paper investigates the attention mechanism of a BERT model that has been trained on protein sequence data and discovers that the language model has implicitly learned non-trivial higher-order biological properties of proteins.
OUTLINE:
0:00 - Intro & Overview
1:40 - From DNA to Proteins
5:20 - BERT for Amino Acid Sequences
8:50 - The Structure of Proteins
12:40 - Investigating Biological Properties by Inspecting BERT
17:45 - Amino Acid Substitution
24:55 - Contact Maps
30:15 - Binding Sites
33:45 - Linear Probes
35:25 - Conclusion & Comments
Paper: https://arxiv.org/abs/2006.15222
Code: https://github.com/salesforce/provis
My Video on BERT: https://youtu.be/-9evrZnBorM
My Video on Attention: https://youtu.be/iDulhoQ2pro
Abstract:
Transformer architectures have proven to learn useful representations for protein classification and generation tasks. However, these representations present challenges in interpretability. Through the lens of attention, we analyze the inner workings of the Transformer and explore how the model discerns structural and functional properties of proteins. We show that attention (1) captures the folding structure of proteins, connecting amino acids that are far apart in the underlying sequence, but spatially close in the three-dimensional structure, (2) targets binding sites, a key functional component of proteins, and (3) focuses on progressively more complex biophysical properties with increasing layer depth. We also present a three-dimensional visualization of the interaction between attention and protein structure. Our findings align with known biological processes and provide a tool to aid discovery in protein engineering and synthetic biology. The code for visualization and analysis is available at this https URL.
Authors: Jesse Vig, Ali Madani, Lav R. Varshney, Caiming Xiong, Richard Socher, Nazneen Fatema Rajani
Links:
YouTube: https://www.youtube.com/c/yannickilcher
Twitter: https://twitter.com/ykilcher
Discord: https://discord.gg/4H8xxDF
BitChute: https://www.bitchute.com/channel/yannic-kilcher
Minds: https://www.minds.com/ykilcher
Видео BERTology Meets Biology: Interpreting Attention in Protein Language Models (Paper Explained) канала Yannic Kilcher
OUTLINE:
0:00 - Intro & Overview
1:40 - From DNA to Proteins
5:20 - BERT for Amino Acid Sequences
8:50 - The Structure of Proteins
12:40 - Investigating Biological Properties by Inspecting BERT
17:45 - Amino Acid Substitution
24:55 - Contact Maps
30:15 - Binding Sites
33:45 - Linear Probes
35:25 - Conclusion & Comments
Paper: https://arxiv.org/abs/2006.15222
Code: https://github.com/salesforce/provis
My Video on BERT: https://youtu.be/-9evrZnBorM
My Video on Attention: https://youtu.be/iDulhoQ2pro
Abstract:
Transformer architectures have proven to learn useful representations for protein classification and generation tasks. However, these representations present challenges in interpretability. Through the lens of attention, we analyze the inner workings of the Transformer and explore how the model discerns structural and functional properties of proteins. We show that attention (1) captures the folding structure of proteins, connecting amino acids that are far apart in the underlying sequence, but spatially close in the three-dimensional structure, (2) targets binding sites, a key functional component of proteins, and (3) focuses on progressively more complex biophysical properties with increasing layer depth. We also present a three-dimensional visualization of the interaction between attention and protein structure. Our findings align with known biological processes and provide a tool to aid discovery in protein engineering and synthetic biology. The code for visualization and analysis is available at this https URL.
Authors: Jesse Vig, Ali Madani, Lav R. Varshney, Caiming Xiong, Richard Socher, Nazneen Fatema Rajani
Links:
YouTube: https://www.youtube.com/c/yannickilcher
Twitter: https://twitter.com/ykilcher
Discord: https://discord.gg/4H8xxDF
BitChute: https://www.bitchute.com/channel/yannic-kilcher
Minds: https://www.minds.com/ykilcher
Видео BERTology Meets Biology: Interpreting Attention in Protein Language Models (Paper Explained) канала Yannic Kilcher
Показать
Комментарии отсутствуют
Информация о видео
Другие видео канала
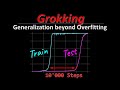
![[ML News] DeepMind does Nowcasting | The Guardian's shady reporting | AI finishes Beethoven's 10th](https://i.ytimg.com/vi/NEkriziVYXo/default.jpg)

![[ML News] Roomba Avoids Poop | Textless NLP | TikTok Algorithm Secrets | New Schmidhuber Blog](https://i.ytimg.com/vi/-sNJd7bANTI/default.jpg)
![[ML News] Plagiarism Case w/ Plot Twist | CLIP for video surveillance | OpenAI summarizes books](https://i.ytimg.com/vi/tX1OolVxDzs/default.jpg)
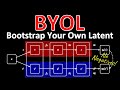

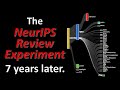
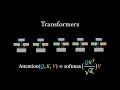
![[ML News] Nvidia renders CEO | Jurassic-1 larger than GPT-3 | Tortured Phrases reveal Plagiarism](https://i.ytimg.com/vi/gu5UM99qaVc/default.jpg)
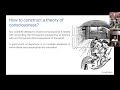
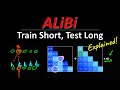
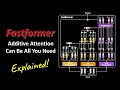
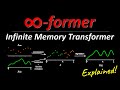
![[ML News] AI predicts race from X-Ray | Google kills HealthStreams | Boosting Search with MuZero](https://i.ytimg.com/vi/eROy3BrqEVk/default.jpg)
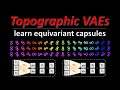
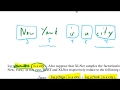

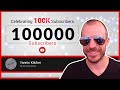
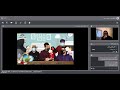