How far can we scale up? Deep Learning's Diminishing Returns (Article Review)
#deeplearning #co2 #cost
Deep Learning has achieved impressive results in the last years, not least due to the massive increases in computational power and data that has gone into these models. Scaling up currently promises to be a reliable way to create more performant systems, but how far can we go? This article explores the limits of exponential scaling in AI, and what people are doing to get around this problem
OUTLINE:
0:00 - Intro & Overview
1:00 - Deep Learning at its limits
3:10 - The cost of overparameterization
5:40 - Extrapolating power usage and CO2 emissions
10:45 - We cannot just continue scaling up
13:25 - Current solution attempts
15:25 - Aside: ImageNet V2
17:50 - Are symbolic methods the way out?
Paper: https://spectrum.ieee.org/deep-learning-computational-cost
Image by Ralf Vetterle from Pixabay: https://pixabay.com/images/id-1752876/
Links:
TabNine Code Completion (Referral): http://bit.ly/tabnine-yannick
YouTube: https://www.youtube.com/c/yannickilcher
Twitter: https://twitter.com/ykilcher
Discord: https://discord.gg/4H8xxDF
BitChute: https://www.bitchute.com/channel/yannic-kilcher
Minds: https://www.minds.com/ykilcher
Parler: https://parler.com/profile/YannicKilcher
LinkedIn: https://www.linkedin.com/in/ykilcher
BiliBili: https://space.bilibili.com/1824646584
If you want to support me, the best thing to do is to share out the content :)
If you want to support me financially (completely optional and voluntary, but a lot of people have asked for this):
SubscribeStar: https://www.subscribestar.com/yannickilcher
Patreon: https://www.patreon.com/yannickilcher
Bitcoin (BTC): bc1q49lsw3q325tr58ygf8sudx2dqfguclvngvy2cq
Ethereum (ETH): 0x7ad3513E3B8f66799f507Aa7874b1B0eBC7F85e2
Litecoin (LTC): LQW2TRyKYetVC8WjFkhpPhtpbDM4Vw7r9m
Monero (XMR): 4ACL8AGrEo5hAir8A9CeVrW8pEauWvnp1WnSDZxW7tziCDLhZAGsgzhRQABDnFy8yuM9fWJDviJPHKRjV4FWt19CJZN9D4n
Видео How far can we scale up? Deep Learning's Diminishing Returns (Article Review) канала Yannic Kilcher
Deep Learning has achieved impressive results in the last years, not least due to the massive increases in computational power and data that has gone into these models. Scaling up currently promises to be a reliable way to create more performant systems, but how far can we go? This article explores the limits of exponential scaling in AI, and what people are doing to get around this problem
OUTLINE:
0:00 - Intro & Overview
1:00 - Deep Learning at its limits
3:10 - The cost of overparameterization
5:40 - Extrapolating power usage and CO2 emissions
10:45 - We cannot just continue scaling up
13:25 - Current solution attempts
15:25 - Aside: ImageNet V2
17:50 - Are symbolic methods the way out?
Paper: https://spectrum.ieee.org/deep-learning-computational-cost
Image by Ralf Vetterle from Pixabay: https://pixabay.com/images/id-1752876/
Links:
TabNine Code Completion (Referral): http://bit.ly/tabnine-yannick
YouTube: https://www.youtube.com/c/yannickilcher
Twitter: https://twitter.com/ykilcher
Discord: https://discord.gg/4H8xxDF
BitChute: https://www.bitchute.com/channel/yannic-kilcher
Minds: https://www.minds.com/ykilcher
Parler: https://parler.com/profile/YannicKilcher
LinkedIn: https://www.linkedin.com/in/ykilcher
BiliBili: https://space.bilibili.com/1824646584
If you want to support me, the best thing to do is to share out the content :)
If you want to support me financially (completely optional and voluntary, but a lot of people have asked for this):
SubscribeStar: https://www.subscribestar.com/yannickilcher
Patreon: https://www.patreon.com/yannickilcher
Bitcoin (BTC): bc1q49lsw3q325tr58ygf8sudx2dqfguclvngvy2cq
Ethereum (ETH): 0x7ad3513E3B8f66799f507Aa7874b1B0eBC7F85e2
Litecoin (LTC): LQW2TRyKYetVC8WjFkhpPhtpbDM4Vw7r9m
Monero (XMR): 4ACL8AGrEo5hAir8A9CeVrW8pEauWvnp1WnSDZxW7tziCDLhZAGsgzhRQABDnFy8yuM9fWJDviJPHKRjV4FWt19CJZN9D4n
Видео How far can we scale up? Deep Learning's Diminishing Returns (Article Review) канала Yannic Kilcher
Показать
Комментарии отсутствуют
Информация о видео
Другие видео канала
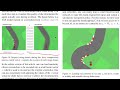
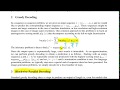
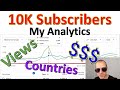
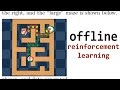
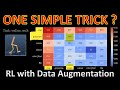
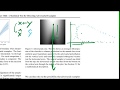

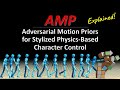
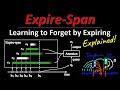
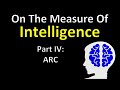
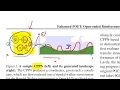
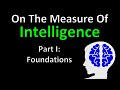
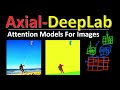
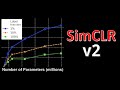
![[Classic] Playing Atari with Deep Reinforcement Learning (Paper Explained)](https://i.ytimg.com/vi/rFwQDDbYTm4/default.jpg)
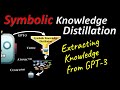
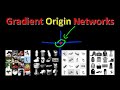
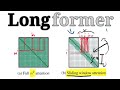
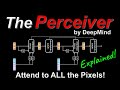
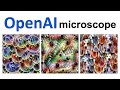
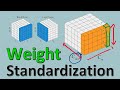