Mihaela van der Schaar: The (Causal) Discovery Ladder: Unravelling Governing Equations and Beyond
- Speaker: Mihaela van der Schaar (University of Cambridge)
- Title: The (Causal) Discovery Ladder: Unravelling Governing Equations and Beyond using Machine Learning
Видео Mihaela van der Schaar: The (Causal) Discovery Ladder: Unravelling Governing Equations and Beyond канала Online Causal Inference Seminar
- Title: The (Causal) Discovery Ladder: Unravelling Governing Equations and Beyond using Machine Learning
Видео Mihaela van der Schaar: The (Causal) Discovery Ladder: Unravelling Governing Equations and Beyond канала Online Causal Inference Seminar
Показать
Комментарии отсутствуют
Информация о видео
17 апреля 2024 г. 21:35:40
00:48:51
Другие видео канала
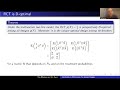
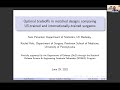
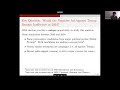
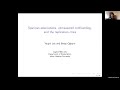
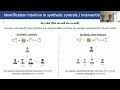
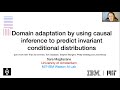
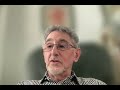
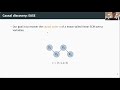
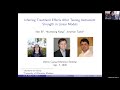
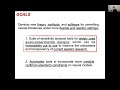
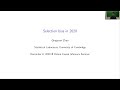
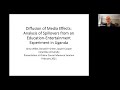

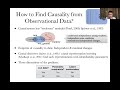
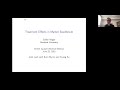

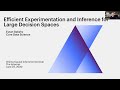
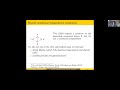
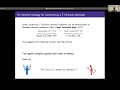
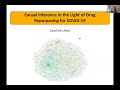
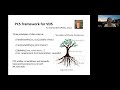