Stefan Wager: Treatment Effects in Market Equilibrium
"Treatment Effects in Market Equilibrium" (joint work with Evan Munro and Kuang Xu)
Stefan Wager, Stanford University
Discussant: Fredrik Sävje, Yale University
Abstract: In order to evaluate social and economic policy, it is important to measure policy effects within a market economy, where individuals interact by buying and selling various goods at the prevailing market price. In this setting, there is a direct policy effect on individual outcomes, and an indirect effect through resulting changes in equilibrium prices, which makes inference through standard randomized trials impossible. We define a stochastic general equilibrium model where interference occurs only through the equilibrium price and find that, in the mean field limit, non-parametrically defined direct and indirect treatment effects of small price changes converge to estimable elasticities with respect to prices and policy. We then show how to design a single-market experiment that enables us to estimate the total policy effect.
June 22, 2021
Видео Stefan Wager: Treatment Effects in Market Equilibrium канала Online Causal Inference Seminar
Stefan Wager, Stanford University
Discussant: Fredrik Sävje, Yale University
Abstract: In order to evaluate social and economic policy, it is important to measure policy effects within a market economy, where individuals interact by buying and selling various goods at the prevailing market price. In this setting, there is a direct policy effect on individual outcomes, and an indirect effect through resulting changes in equilibrium prices, which makes inference through standard randomized trials impossible. We define a stochastic general equilibrium model where interference occurs only through the equilibrium price and find that, in the mean field limit, non-parametrically defined direct and indirect treatment effects of small price changes converge to estimable elasticities with respect to prices and policy. We then show how to design a single-market experiment that enables us to estimate the total policy effect.
June 22, 2021
Видео Stefan Wager: Treatment Effects in Market Equilibrium канала Online Causal Inference Seminar
Показать
Комментарии отсутствуют
Информация о видео
25 июня 2021 г. 11:43:47
01:03:08
Другие видео канала
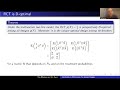
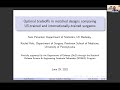
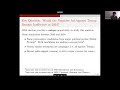
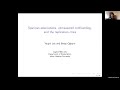
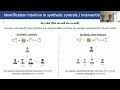
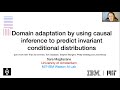
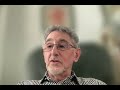
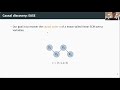
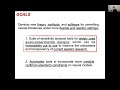
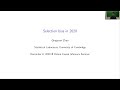
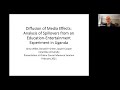

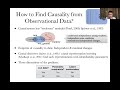

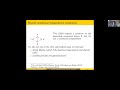
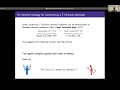
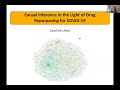
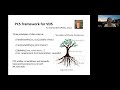
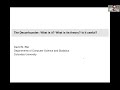
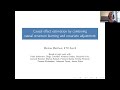