Bin Yu: Predictability, stability, and causality with case study of genetic drivers of heart disease
- Speaker: Bin Yu (UC Berkeley)
- Title: Predictability, stability, and causality with a case study to find genetic drivers of a heart disease
- Discussant: Jas Sekhon (Yale University)
- Abstract: "A.I. is like nuclear energy -- both promising and dangerous" -- Bill Gates, 2019.
Data Science is a pillar of A.I. and has driven most of recent cutting-edge discoveries in biomedical research and beyond. Human judgement calls are ubiquitous at every step of a data science life cycle, e.g., in choosing data cleaning methods, predictive algorithms and data perturbations. Such judgment calls are often responsible for the "dangers" of A.I. To maximally mitigate these dangers, we developed a framework based on three core principles: Predictability, Computability and Stability (PCS). The PCS framework unifies and expands on the best practices of machine learning and statistics. It consists of a workflow and documentation and is supported by our software package v-flow.In this talk, we first illustrate the PCS framework through the development of iterative random forests (iRF) for predictable and stable non-linear interaction discovery (in collaboration with the Brown Lab at LBNL and Berkeley Statistics). In pursuit of genetic drivers of a heart disease called hypertrophic cardiomyopathy (HCM) as a CZ Biohub project in collaboration with the Ashley Lab at Stanford Medical School and others, we use iRF and UK Biobank data to recommend gene-gene interaction targets for knock-down experiments. We then analyze the experimental data to show promising findings about genetic drivers of HCM.
Видео Bin Yu: Predictability, stability, and causality with case study of genetic drivers of heart disease канала Online Causal Inference Seminar
- Title: Predictability, stability, and causality with a case study to find genetic drivers of a heart disease
- Discussant: Jas Sekhon (Yale University)
- Abstract: "A.I. is like nuclear energy -- both promising and dangerous" -- Bill Gates, 2019.
Data Science is a pillar of A.I. and has driven most of recent cutting-edge discoveries in biomedical research and beyond. Human judgement calls are ubiquitous at every step of a data science life cycle, e.g., in choosing data cleaning methods, predictive algorithms and data perturbations. Such judgment calls are often responsible for the "dangers" of A.I. To maximally mitigate these dangers, we developed a framework based on three core principles: Predictability, Computability and Stability (PCS). The PCS framework unifies and expands on the best practices of machine learning and statistics. It consists of a workflow and documentation and is supported by our software package v-flow.In this talk, we first illustrate the PCS framework through the development of iterative random forests (iRF) for predictable and stable non-linear interaction discovery (in collaboration with the Brown Lab at LBNL and Berkeley Statistics). In pursuit of genetic drivers of a heart disease called hypertrophic cardiomyopathy (HCM) as a CZ Biohub project in collaboration with the Ashley Lab at Stanford Medical School and others, we use iRF and UK Biobank data to recommend gene-gene interaction targets for knock-down experiments. We then analyze the experimental data to show promising findings about genetic drivers of HCM.
Видео Bin Yu: Predictability, stability, and causality with case study of genetic drivers of heart disease канала Online Causal Inference Seminar
Показать
Комментарии отсутствуют
Информация о видео
1 июня 2022 г. 22:11:31
01:04:26
Другие видео канала
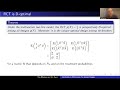
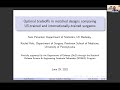
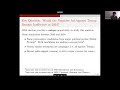
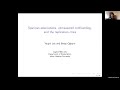
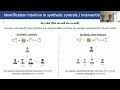
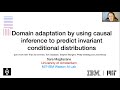
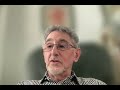
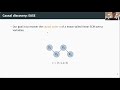
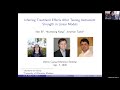
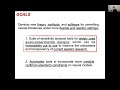
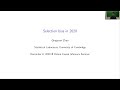
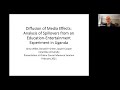

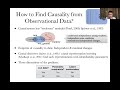
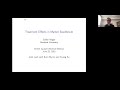
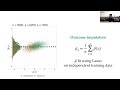
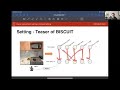
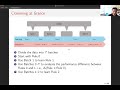
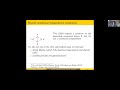
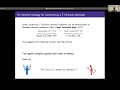
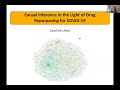