Backpropagation (Part 4): The Sigmoid Transfer Function and Its Derivative
A differentiable transfer function, such as the sigmoid (logistic) function, is essential for the backpropagation training method for neural networks such as the Multilayer Perceptron (MLP). After re-acquainting ourselves with the chain rule from differential calculus (in vids 3a and 3b), we now apply the chain rule to taking the derivative of the transfer function with respect to the node inputs into that function.
Видео Backpropagation (Part 4): The Sigmoid Transfer Function and Its Derivative канала Alianna J. Maren
Видео Backpropagation (Part 4): The Sigmoid Transfer Function and Its Derivative канала Alianna J. Maren
Показать
Комментарии отсутствуют
Информация о видео
Другие видео канала
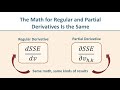
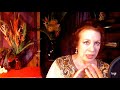
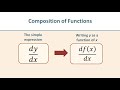
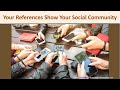
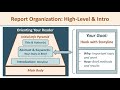
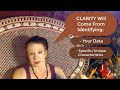
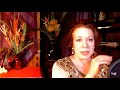
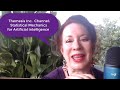


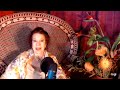
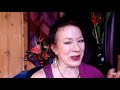
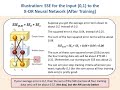

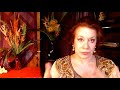
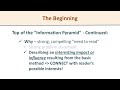

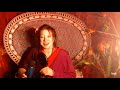
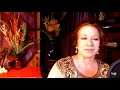
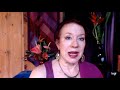