Tess Smidt - Symmetry’s made to be broken: Learning how to break symmetry with symmetry-preservin...
Prof. Tess Smidt of the MIT speaking in the UW Data-driven methods in science and engineering seminar on January 6, 2023.
Sign up for notifications of future talks: https://mailman11.u.washington.edu/mailman/listinfo/datadriven-seminar
Title: Symmetry’s made to be broken: Learning how to break symmetry with symmetry-preserving neural networks
Abstract: Symmetry-preserving (equivariant) neural networks are extremely data-efficient and generalize well when applied to diverse domains (e.g. computer vision and atomic systems). But, are there circumstances where equivariance is too strict or otherwise undesirable? In this talk, I’ll discuss how symmetry-preserving neural networks can learn symmetry-breaking information in order to fit a dataset that may have missing information unbeknownst to the researcher. Furthermore, due to the mathematical guarantees of equivariant neural networks, these learned parameters are guaranteed to be minimally symmetry breaking. I’ll describe network architectures that can learn these symmetry-breaking parameters in two distinct settings: 1) symmetry-breaking parameters are learned for an entire dataset to capture global asymmetries in the data and 2) symmetry-breaking parameters are predicted in an equivariant manner for individual examples. Finally, I’ll demonstrate these networks on several prototypical examples and apply them to predicting structural distortions of crystalline materials.
Видео Tess Smidt - Symmetry’s made to be broken: Learning how to break symmetry with symmetry-preservin... канала Physics Informed Machine Learning
Sign up for notifications of future talks: https://mailman11.u.washington.edu/mailman/listinfo/datadriven-seminar
Title: Symmetry’s made to be broken: Learning how to break symmetry with symmetry-preserving neural networks
Abstract: Symmetry-preserving (equivariant) neural networks are extremely data-efficient and generalize well when applied to diverse domains (e.g. computer vision and atomic systems). But, are there circumstances where equivariance is too strict or otherwise undesirable? In this talk, I’ll discuss how symmetry-preserving neural networks can learn symmetry-breaking information in order to fit a dataset that may have missing information unbeknownst to the researcher. Furthermore, due to the mathematical guarantees of equivariant neural networks, these learned parameters are guaranteed to be minimally symmetry breaking. I’ll describe network architectures that can learn these symmetry-breaking parameters in two distinct settings: 1) symmetry-breaking parameters are learned for an entire dataset to capture global asymmetries in the data and 2) symmetry-breaking parameters are predicted in an equivariant manner for individual examples. Finally, I’ll demonstrate these networks on several prototypical examples and apply them to predicting structural distortions of crystalline materials.
Видео Tess Smidt - Symmetry’s made to be broken: Learning how to break symmetry with symmetry-preservin... канала Physics Informed Machine Learning
Показать
Комментарии отсутствуют
Информация о видео
3 февраля 2023 г. 4:04:23
01:04:15
Другие видео канала
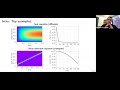
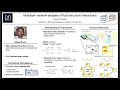
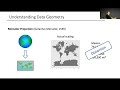
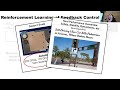
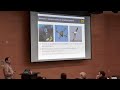
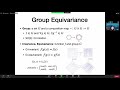
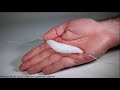


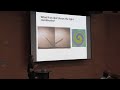
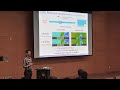

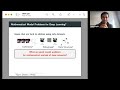
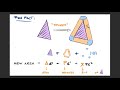
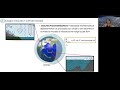
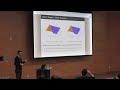
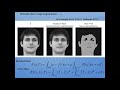
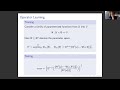
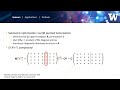
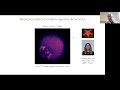
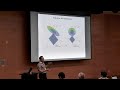