John Wright - Deep Networks and the Multiple Manifold Problem
Prof. John Wright of Columbia University speaking in the UW Data-driven methods in science and engineering seminar on October 7, 2022.
Sign up for notifications of future talks: https://mailman11.u.washington.edu/mailman/listinfo/datadriven-seminar
Abstract: Data in science and engineering often exhibit nonlinear, low-dimensional structure, due to the physical laws that govern data generation. In this talk, we study how deep neural networks interact with structured data:
+ When can we guarantee to fit and generalize?
+ How do the resources (depth, width, data) required depend on the complexity of the data?
+ How can we leverage physical prior knowledge to reduce these resource requirements?
Our main mathematical result is a guarantee of generalization for a model classification problem involving data on low-dimensional manifolds — we prove that for networks of polynomial width, with polynomially many samples, randomly initialized gradient descent rapidly converges to a solution which correctly labels *every* point on the two manifolds. To our knowledge this is the first such result for deep networks on data which are not linearly separable. We highlight intuitions about the roles of depth, width, sample complexity, and the geometry of feature representations, which may be useful in analyzing other problems involving low-dimensional structure (e.g., model discovery). We illustrate these ideas through applied problems in astrophysics and computer vision. In these settings, we further suggest how incorporating physical prior knowledge can reduce the resources (architecture, data) required for learning, leading to more efficient and interpretable learning architectures.
Видео John Wright - Deep Networks and the Multiple Manifold Problem канала Physics Informed Machine Learning
Sign up for notifications of future talks: https://mailman11.u.washington.edu/mailman/listinfo/datadriven-seminar
Abstract: Data in science and engineering often exhibit nonlinear, low-dimensional structure, due to the physical laws that govern data generation. In this talk, we study how deep neural networks interact with structured data:
+ When can we guarantee to fit and generalize?
+ How do the resources (depth, width, data) required depend on the complexity of the data?
+ How can we leverage physical prior knowledge to reduce these resource requirements?
Our main mathematical result is a guarantee of generalization for a model classification problem involving data on low-dimensional manifolds — we prove that for networks of polynomial width, with polynomially many samples, randomly initialized gradient descent rapidly converges to a solution which correctly labels *every* point on the two manifolds. To our knowledge this is the first such result for deep networks on data which are not linearly separable. We highlight intuitions about the roles of depth, width, sample complexity, and the geometry of feature representations, which may be useful in analyzing other problems involving low-dimensional structure (e.g., model discovery). We illustrate these ideas through applied problems in astrophysics and computer vision. In these settings, we further suggest how incorporating physical prior knowledge can reduce the resources (architecture, data) required for learning, leading to more efficient and interpretable learning architectures.
Видео John Wright - Deep Networks and the Multiple Manifold Problem канала Physics Informed Machine Learning
Показать
Комментарии отсутствуют
Информация о видео
21 октября 2022 г. 22:01:28
01:08:19
Другие видео канала
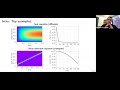
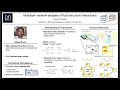
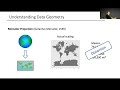
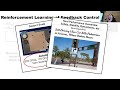
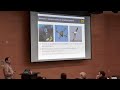
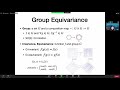
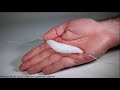


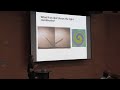
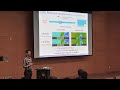

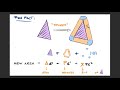
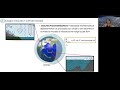
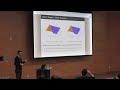
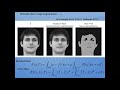
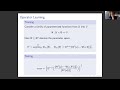
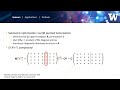
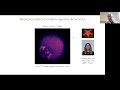
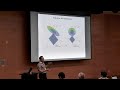