Beverley McKeon - What’s in a mean (what, how and why)? Towards nonlinear models of wall turbulence
Talk starts at 2:00
Dr. Beverley McKeon from Caltech speaking in the Data-Driven Methods for Science and Engineering Seminar on February 19, 2021
For more information including past and upcoming talks, visit: http://www.databookuw.com/seminars/
Sign up for notifications of future talks: https://mailman.u.washington.edu/mailman/listinfo/datadriven-seminar
Abstract:
In this talk I will discuss the fusion of analytical and data-based approaches to understanding the nonlinear interactions sustaining turbulence near a wall. Exploiting information embedded in the turbulent mean field (and how it gets there) using resolvent analysis, emphasis will be placed on discovering strict rules governing the important scale-to-scale interactions. By consideration of a range of flow configurations, a dramatic reduction in complexity of these problems will be demonstrated, associated with sparsity, self-similarity and low-rank behavior in the resolvent (attributable to the information encoded in the turbulent mean field). In this talk, we will synthesize data and classical analysis techniques to distill clues guiding reconstruction of the full nonlinear flow field from the most amplified spatio-temporal modes, and the associated characteristics of the mean field.
The support of the U.S. Air Force Office of Scientific Research under grant FA 9550-16-1-0361 and the U.S. Office of Naval Research under grants N00014-17-1-2307 & N00014-17-1-3022 is gratefully acknowledged.
Видео Beverley McKeon - What’s in a mean (what, how and why)? Towards nonlinear models of wall turbulence канала Physics Informed Machine Learning
Dr. Beverley McKeon from Caltech speaking in the Data-Driven Methods for Science and Engineering Seminar on February 19, 2021
For more information including past and upcoming talks, visit: http://www.databookuw.com/seminars/
Sign up for notifications of future talks: https://mailman.u.washington.edu/mailman/listinfo/datadriven-seminar
Abstract:
In this talk I will discuss the fusion of analytical and data-based approaches to understanding the nonlinear interactions sustaining turbulence near a wall. Exploiting information embedded in the turbulent mean field (and how it gets there) using resolvent analysis, emphasis will be placed on discovering strict rules governing the important scale-to-scale interactions. By consideration of a range of flow configurations, a dramatic reduction in complexity of these problems will be demonstrated, associated with sparsity, self-similarity and low-rank behavior in the resolvent (attributable to the information encoded in the turbulent mean field). In this talk, we will synthesize data and classical analysis techniques to distill clues guiding reconstruction of the full nonlinear flow field from the most amplified spatio-temporal modes, and the associated characteristics of the mean field.
The support of the U.S. Air Force Office of Scientific Research under grant FA 9550-16-1-0361 and the U.S. Office of Naval Research under grants N00014-17-1-2307 & N00014-17-1-3022 is gratefully acknowledged.
Видео Beverley McKeon - What’s in a mean (what, how and why)? Towards nonlinear models of wall turbulence канала Physics Informed Machine Learning
Показать
Комментарии отсутствуют
Информация о видео
20 февраля 2021 г. 0:01:07
00:53:10
Другие видео канала
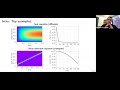
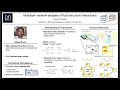
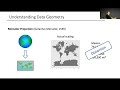
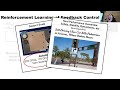
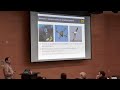
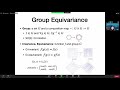
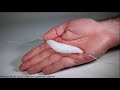


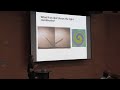
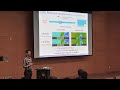

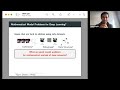
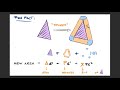
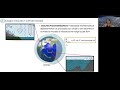
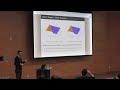
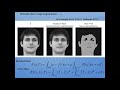
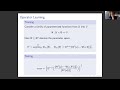
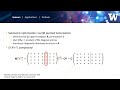
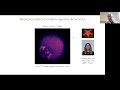
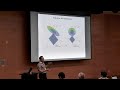