[Live Machine Learning Research] Plain Self-Ensembles (I actually DISCOVER SOMETHING) - Part 1
I share my progress of implementing a research idea from scratch. I attempt to build an ensemble model out of students of label-free self-distillation without any additional data or augmentation. Turns out, it actually works, and interestingly, the more students I employ, the better the accuracy. This leads to the hypothesis that the ensemble effect is not a process of extracting more information from labels.
OUTLINE:
0:00 - Introduction
2:10 - Research Idea
4:15 - Adjusting the Codebase
25:00 - Teacher and Student Models
52:30 - Shipping to the Server
1:03:40 - Results
1:14:50 - Conclusion
Code: https://github.com/yk/PyTorch_CIFAR10
References:
My Video on SimCLRv2: https://youtu.be/2lkUNDZld-4
Born-Again Neural Networks: https://arxiv.org/abs/1805.04770
Deep Ensembles: A Loss Landscape Perspective: https://arxiv.org/abs/1912.02757
Links:
YouTube: https://www.youtube.com/c/yannickilcher
Twitter: https://twitter.com/ykilcher
Discord: https://discord.gg/4H8xxDF
BitChute: https://www.bitchute.com/channel/yannic-kilcher
Minds: https://www.minds.com/ykilcher
Parler: https://parler.com/profile/YannicKilcher
Видео [Live Machine Learning Research] Plain Self-Ensembles (I actually DISCOVER SOMETHING) - Part 1 канала Yannic Kilcher
OUTLINE:
0:00 - Introduction
2:10 - Research Idea
4:15 - Adjusting the Codebase
25:00 - Teacher and Student Models
52:30 - Shipping to the Server
1:03:40 - Results
1:14:50 - Conclusion
Code: https://github.com/yk/PyTorch_CIFAR10
References:
My Video on SimCLRv2: https://youtu.be/2lkUNDZld-4
Born-Again Neural Networks: https://arxiv.org/abs/1805.04770
Deep Ensembles: A Loss Landscape Perspective: https://arxiv.org/abs/1912.02757
Links:
YouTube: https://www.youtube.com/c/yannickilcher
Twitter: https://twitter.com/ykilcher
Discord: https://discord.gg/4H8xxDF
BitChute: https://www.bitchute.com/channel/yannic-kilcher
Minds: https://www.minds.com/ykilcher
Parler: https://parler.com/profile/YannicKilcher
Видео [Live Machine Learning Research] Plain Self-Ensembles (I actually DISCOVER SOMETHING) - Part 1 канала Yannic Kilcher
Показать
Комментарии отсутствуют
Информация о видео
Другие видео канала
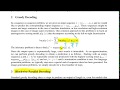
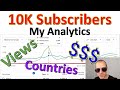
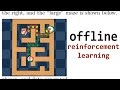
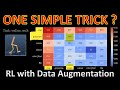
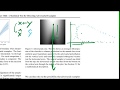

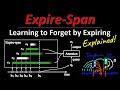
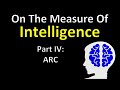
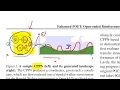
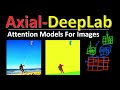
![[Classic] Playing Atari with Deep Reinforcement Learning (Paper Explained)](https://i.ytimg.com/vi/rFwQDDbYTm4/default.jpg)
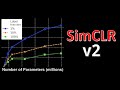
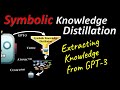
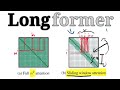
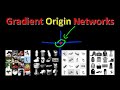
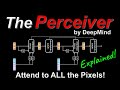
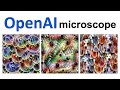
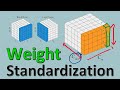
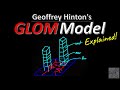
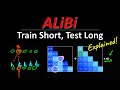
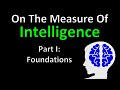