Arthur Gretton - Generalized Energy-Based Models
Abstract: I will introduce Generalized Energy Based Models (GEBM) for generative modelling. These models combine two trained components: a base distribution (generally an implicit model), which can learn the support of data with low intrinsic dimension in a high dimensional space; and an energy function, to refine the probability mass on the learned support. Both the energy function and base jointly constitute the final model, unlike GANs, which retain only the base distribution (the "generator"). In particular, while the energy function is analogous to the GAN critic function, it is not discarded after training. GEBMs are trained by alternating between learning the energy and the base. We show that both training stages are well-defined: the energy is learned by maximising a generalized likelihood, and the resulting energy-based loss provides informative gradients for learning the base. Samples from the posterior on the latent space of the trained model can be obtained via MCMC, thus finding regions in this space that produce better quality samples. Empirically, the GEBM samples on image-generation tasks are of much better quality than those from the learned generator alone, indicating that all else being equal, the GEBM will outperform a GAN of the same complexity. GEBMs also return state-of-the-art performance on density modelling tasks, and when using base measures with an explicit form.
Speaker: Arthur Gretton is a Professor at the Gatsby Computational Neuroscience Unit and Director of the Centre for Computational Statistics and Machine Learning, at University College London. His personal website can be found at http://www.gatsby.ucl.ac.uk/~gretton/.
This talk was given at Secondmind Labs, as a part of our (virtual) research seminar. Our research seminar is where we exchange ideas with guest speakers, keeping you up to date with the latest developments and inspiring research topics. Occasionally, Secondmind researchers present their own work as well. You can find a complete list of speakers at https://www.secondmind.ai/labs/seminars/. Learn more about Secondmind Labs at https://www.secondmind.ai/labs/
Видео Arthur Gretton - Generalized Energy-Based Models канала Secondmind
Speaker: Arthur Gretton is a Professor at the Gatsby Computational Neuroscience Unit and Director of the Centre for Computational Statistics and Machine Learning, at University College London. His personal website can be found at http://www.gatsby.ucl.ac.uk/~gretton/.
This talk was given at Secondmind Labs, as a part of our (virtual) research seminar. Our research seminar is where we exchange ideas with guest speakers, keeping you up to date with the latest developments and inspiring research topics. Occasionally, Secondmind researchers present their own work as well. You can find a complete list of speakers at https://www.secondmind.ai/labs/seminars/. Learn more about Secondmind Labs at https://www.secondmind.ai/labs/
Видео Arthur Gretton - Generalized Energy-Based Models канала Secondmind
Показать
Комментарии отсутствуют
Информация о видео
Другие видео канала
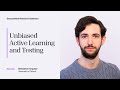
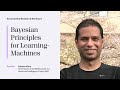
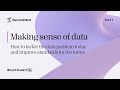
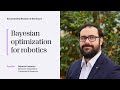
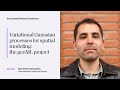
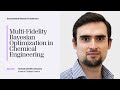
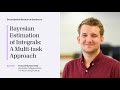
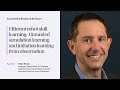
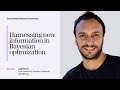
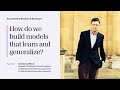
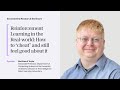
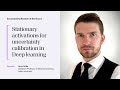
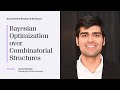

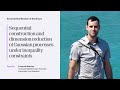
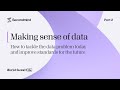

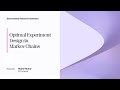
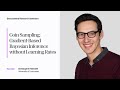
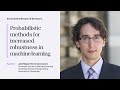
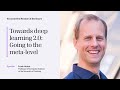