Vincent Adam - Sparse methods for markovian GPs
Abstract:
Gaussian Processes (GP) provide rich priors for time series models. Markovian GPs with 1d input have an equivalent representation as stochastic differential equations (SDE) whose structure allows for the derivation of fast (approximate) inference algorithms. Their typical computational complexity scales linearly with the number of data points O(N), with computations inherently sequential. Using inducing states of this SDE to support a sparse GP approximation to the posterior process leads to further computational savings by making the O(N) scaling parallel. I will present various approximate inference algorithms based on this sparse approximation including Laplace, expectation-propagation and variational inference and I will discuss their performance guarantees and comparative advantages.
Speaker: Vincent Adam is a Senior Machine Learning Researcher at Secondmind.ai, and Postdoctoral researcher at Aalto University, Finland. More info can be found at the personal website: https://vincentadam87.github.io/
This talk was given at Secondmind Labs, as a part of our (virtual) research seminar. Our research seminar is where we exchange ideas with guest speakers, keeping you up to date with the latest developments and inspiring research topics. Occasionally, Secondmind researchers present their own work as well. You can find a complete list of speakers at https://www.secondmind.ai/labs/seminars/. Learn more about Secondmind Labs at https://www.secondmind.ai/labs/
Видео Vincent Adam - Sparse methods for markovian GPs канала Secondmind
Gaussian Processes (GP) provide rich priors for time series models. Markovian GPs with 1d input have an equivalent representation as stochastic differential equations (SDE) whose structure allows for the derivation of fast (approximate) inference algorithms. Their typical computational complexity scales linearly with the number of data points O(N), with computations inherently sequential. Using inducing states of this SDE to support a sparse GP approximation to the posterior process leads to further computational savings by making the O(N) scaling parallel. I will present various approximate inference algorithms based on this sparse approximation including Laplace, expectation-propagation and variational inference and I will discuss their performance guarantees and comparative advantages.
Speaker: Vincent Adam is a Senior Machine Learning Researcher at Secondmind.ai, and Postdoctoral researcher at Aalto University, Finland. More info can be found at the personal website: https://vincentadam87.github.io/
This talk was given at Secondmind Labs, as a part of our (virtual) research seminar. Our research seminar is where we exchange ideas with guest speakers, keeping you up to date with the latest developments and inspiring research topics. Occasionally, Secondmind researchers present their own work as well. You can find a complete list of speakers at https://www.secondmind.ai/labs/seminars/. Learn more about Secondmind Labs at https://www.secondmind.ai/labs/
Видео Vincent Adam - Sparse methods for markovian GPs канала Secondmind
Показать
Комментарии отсутствуют
Информация о видео
Другие видео канала
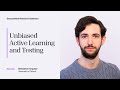
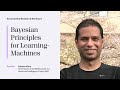
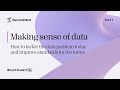
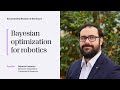
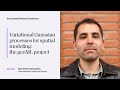
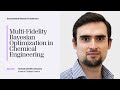
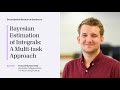
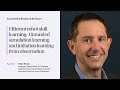
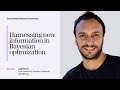
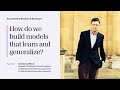
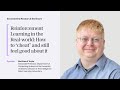
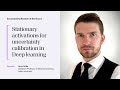
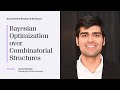
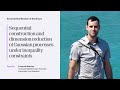
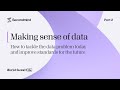

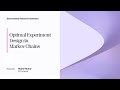
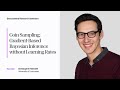
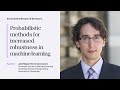
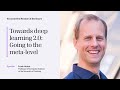