Weaving together machine learning, theoretical physics, and neuroscience by Surya Ganguli
Surya Ganguli (Stanford University), "Weaving together machine learning, theoretical physics, and neuroscience"
https://ganguli-gang.stanford.edu/
Abstract: An exciting area of intellectual activity in this century may well revolve around a synthesis of machine learning, theoretical physics, and neuroscience. The unification of these fields will likely enable us to exploit the power of complex systems analysis, developed in theoretical physics and applied mathematics, to elucidate the design principles governing neural systems, both biological and artificial, and deploy these principles to develop better algorithms in machine learning. We will give several vignettes in this direction, including: (1) determining the best optimization problem to solve in order to perform regression in high dimensions; (2) finding exact solutions to the dynamics of generalization error in deep linear networks; (3) developing interpretable machine learning to derive and understand state of the art models of the retina; (4) analyzing and explaining the origins of hexagonal firing patterns in recurrent neural networks trained to path-integrate; (5) understanding the geometry and dynamics of high dimensional optimization in the classical limit of dissipative many-body quantum optimizers.
References:
M. Advani and S. Ganguli, Statistical mechanics of optimal convex inference in high dimensions, Physical Review X, 6, 031034, 2016.
M. Advani and S. Ganguli, An equivalence between high dimensional Bayes optimal inference and M-estimation, NeurIPS, 2016.
A.K. Lampinen and S. Ganguli, An analytic theory of generalization dynamics and transfer learning in deep linear networks, International Conference on Learning Representations (ICLR), 2019.
H. Tanaka, A. Nayebi, N. Maheswaranathan, L.M. McIntosh, S. Baccus, S. Ganguli, From deep learning to mechanistic understanding in neuroscience: the structure of retinal prediction, NeurIPS 2019.
S. Deny, J. Lindsey, S. Ganguli, S. Ocko, The emergence of multiple retinal cell types through efficient coding of natural movies, Neural Information Processing Systems (NeurIPS) 2018.
B. Sorscher, G. Mel, S. Ganguli, S. Ocko, A unified theory for the origin of grid cells through the lens of pattern formation, NeurIPS 2019.
Y. Bahri, J. Kadmon, J. Pennington, S. Schoenholz, J. Sohl-Dickstein, and S. Ganguli, Statistical mechanics of deep learning, Annual Reviews of Condensed Matter Physics, 2020.
Y. Yamamoto, T. Leleu, S. Ganguli and H. Mabuchi, Coherent Ising Machines: quantum optics and neural network perspectives, Applied Physics Letters 2020.
B.P. Marsh, Y, Guo, R.M. Kroeze, S. Gopalakrishnan, S. Ganguli, J. Keeling, B.L. Lev, Enhancing associative memory recall and storage capacity using confocal cavity QED, https://arxiv.org/abs/2009.01227
AAAI 2021 Spring Symposium on Combining Artificial Intelligence and Machine Learning with Physics Sciences, March 22-24, 2021 (https://sites.google.com/view/aaai-mlps)
Papers: https://sites.google.com/view/aaai-mlps/proceedings
Slides: https://sites.google.com/view/aaai-mlps/program
Видео Weaving together machine learning, theoretical physics, and neuroscience by Surya Ganguli канала MLPS - Combining AI and ML with Physics Sciences
https://ganguli-gang.stanford.edu/
Abstract: An exciting area of intellectual activity in this century may well revolve around a synthesis of machine learning, theoretical physics, and neuroscience. The unification of these fields will likely enable us to exploit the power of complex systems analysis, developed in theoretical physics and applied mathematics, to elucidate the design principles governing neural systems, both biological and artificial, and deploy these principles to develop better algorithms in machine learning. We will give several vignettes in this direction, including: (1) determining the best optimization problem to solve in order to perform regression in high dimensions; (2) finding exact solutions to the dynamics of generalization error in deep linear networks; (3) developing interpretable machine learning to derive and understand state of the art models of the retina; (4) analyzing and explaining the origins of hexagonal firing patterns in recurrent neural networks trained to path-integrate; (5) understanding the geometry and dynamics of high dimensional optimization in the classical limit of dissipative many-body quantum optimizers.
References:
M. Advani and S. Ganguli, Statistical mechanics of optimal convex inference in high dimensions, Physical Review X, 6, 031034, 2016.
M. Advani and S. Ganguli, An equivalence between high dimensional Bayes optimal inference and M-estimation, NeurIPS, 2016.
A.K. Lampinen and S. Ganguli, An analytic theory of generalization dynamics and transfer learning in deep linear networks, International Conference on Learning Representations (ICLR), 2019.
H. Tanaka, A. Nayebi, N. Maheswaranathan, L.M. McIntosh, S. Baccus, S. Ganguli, From deep learning to mechanistic understanding in neuroscience: the structure of retinal prediction, NeurIPS 2019.
S. Deny, J. Lindsey, S. Ganguli, S. Ocko, The emergence of multiple retinal cell types through efficient coding of natural movies, Neural Information Processing Systems (NeurIPS) 2018.
B. Sorscher, G. Mel, S. Ganguli, S. Ocko, A unified theory for the origin of grid cells through the lens of pattern formation, NeurIPS 2019.
Y. Bahri, J. Kadmon, J. Pennington, S. Schoenholz, J. Sohl-Dickstein, and S. Ganguli, Statistical mechanics of deep learning, Annual Reviews of Condensed Matter Physics, 2020.
Y. Yamamoto, T. Leleu, S. Ganguli and H. Mabuchi, Coherent Ising Machines: quantum optics and neural network perspectives, Applied Physics Letters 2020.
B.P. Marsh, Y, Guo, R.M. Kroeze, S. Gopalakrishnan, S. Ganguli, J. Keeling, B.L. Lev, Enhancing associative memory recall and storage capacity using confocal cavity QED, https://arxiv.org/abs/2009.01227
AAAI 2021 Spring Symposium on Combining Artificial Intelligence and Machine Learning with Physics Sciences, March 22-24, 2021 (https://sites.google.com/view/aaai-mlps)
Papers: https://sites.google.com/view/aaai-mlps/proceedings
Slides: https://sites.google.com/view/aaai-mlps/program
Видео Weaving together machine learning, theoretical physics, and neuroscience by Surya Ganguli канала MLPS - Combining AI and ML with Physics Sciences
Показать
Комментарии отсутствуют
Информация о видео
13 апреля 2021 г. 6:47:09
01:05:26
Другие видео канала
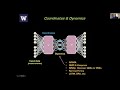
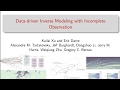

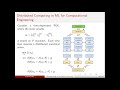
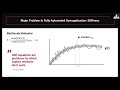
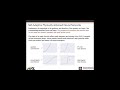
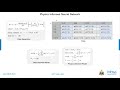

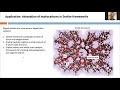

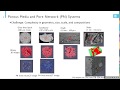
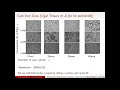
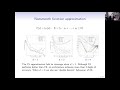
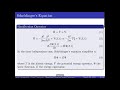
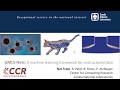
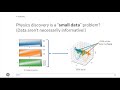
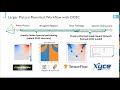

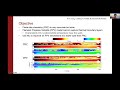
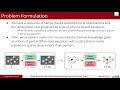
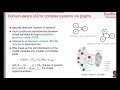