Deep learning for scientific computing: (closing) the gap between theory and practice by Ben Adcock
Ben Adcock (Simon Fraser University), "Deep learning for scientific computing: (closing) the gap between theory and practice"
https://benadcock.org/
Abstract: Deep learning is starting to be increasingly used for challenging problems in scientific computing. Theoretically, such efforts are supported by a large and growing body of literature on existence of deep neural networks with favourable approximation properties. Yet, these results often say very little about practical performance in terms of the traditional pillars of numerical analysis: accuracy, stability, sampling complexity and computational cost. In this talk, I will focus on two distinct problems in scientific computing to which deep learning is being actively applied: high-dimensional function approximation and inverse problems for imaging. In each case, I will first highlight several limitations of current approaches in terms of stability, unpredictable generalization and/or the gap between existence theory and practical performance. Then, I will showcase recent theoretical contributions that show that deep neural networks matching the performance of best-in-class schemes can be computed in both settings. This highlights the potential of deep neural networks, and sheds light on achieving robust, reliable and overall improved practical performance.
References:
B. Adcock, S. Brugiapaglia, N. Dexter & S. Moraga, Deep neural networks are effective at learning high-dimensional Hilbert-valued functions from limited data, MSML21 (in revision), 2021.
B. Adcock & N. Dexter, The gap between theory and practice in function approximation with deep neural networks, SIAM J. Math. Data Sci. (to appear), 2021.
B. Adcock & A. C. Hansen. Compressive Imaging: Structure, Sampling, Learning, CUP (in press), 2021.
V. Antun, F. Renna, C. Poon, B. Adcock & A. C. Hansen, On instabilities of deep learning in image reconstruction and the potential costs of AI, Proc. Natl. Acad. Sci. USA 117(48):30088--30095, 2020.
AAAI 2021 Spring Symposium on Combining Artificial Intelligence and Machine Learning with Physics Sciences, March 22-24, 2021 (https://sites.google.com/view/aaai-mlps)
Papers: https://sites.google.com/view/aaai-mlps/proceedings
Slides: https://sites.google.com/view/aaai-mlps/program
Видео Deep learning for scientific computing: (closing) the gap between theory and practice by Ben Adcock канала MLPS - Combining AI and ML with Physics Sciences
https://benadcock.org/
Abstract: Deep learning is starting to be increasingly used for challenging problems in scientific computing. Theoretically, such efforts are supported by a large and growing body of literature on existence of deep neural networks with favourable approximation properties. Yet, these results often say very little about practical performance in terms of the traditional pillars of numerical analysis: accuracy, stability, sampling complexity and computational cost. In this talk, I will focus on two distinct problems in scientific computing to which deep learning is being actively applied: high-dimensional function approximation and inverse problems for imaging. In each case, I will first highlight several limitations of current approaches in terms of stability, unpredictable generalization and/or the gap between existence theory and practical performance. Then, I will showcase recent theoretical contributions that show that deep neural networks matching the performance of best-in-class schemes can be computed in both settings. This highlights the potential of deep neural networks, and sheds light on achieving robust, reliable and overall improved practical performance.
References:
B. Adcock, S. Brugiapaglia, N. Dexter & S. Moraga, Deep neural networks are effective at learning high-dimensional Hilbert-valued functions from limited data, MSML21 (in revision), 2021.
B. Adcock & N. Dexter, The gap between theory and practice in function approximation with deep neural networks, SIAM J. Math. Data Sci. (to appear), 2021.
B. Adcock & A. C. Hansen. Compressive Imaging: Structure, Sampling, Learning, CUP (in press), 2021.
V. Antun, F. Renna, C. Poon, B. Adcock & A. C. Hansen, On instabilities of deep learning in image reconstruction and the potential costs of AI, Proc. Natl. Acad. Sci. USA 117(48):30088--30095, 2020.
AAAI 2021 Spring Symposium on Combining Artificial Intelligence and Machine Learning with Physics Sciences, March 22-24, 2021 (https://sites.google.com/view/aaai-mlps)
Papers: https://sites.google.com/view/aaai-mlps/proceedings
Slides: https://sites.google.com/view/aaai-mlps/program
Видео Deep learning for scientific computing: (closing) the gap between theory and practice by Ben Adcock канала MLPS - Combining AI and ML with Physics Sciences
Показать
Комментарии отсутствуют
Информация о видео
13 апреля 2021 г. 6:52:10
01:09:50
Другие видео канала
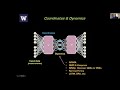
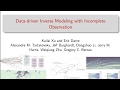

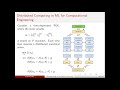
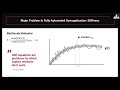
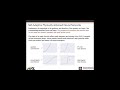
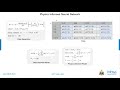

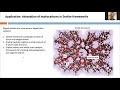

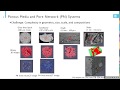
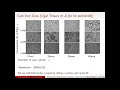
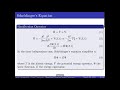
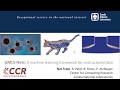
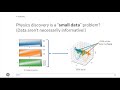
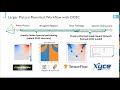

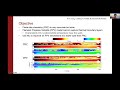
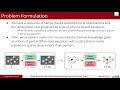
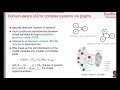