Dimensionality Reduction and Clustering Tools for High Parameter Discovery
Originally filmed on 9/19/19
In this webinar Application Scientist, Dr. Timothy Quinn Crawford explores Dimensionality Reduction and Clustering through FlowJo and SeqGeq plugins.
Dimensionality Reduction has become a popular way of visualizing high parameter datasets and is useful in overcoming some of the major obstacles associated with this type of data. The goal in dimensionality reduction is to reduce the number of variables under consideration (i.e., gene reads) and to obtain a set of principal variables (i.e., analytical parameters). This is particularly useful as working with too many dimensions would be overwhelming and one needs some help to visualize this amount of information. Dimensionality reduction takes your high dimensional dataset and allows researchers to view it on a biaxial plot while preserving variance contained in biological populations.
You can download plugins for yourself on FlowJo Exchange: https://www.flowjo.com/exchange/#/
Видео Dimensionality Reduction and Clustering Tools for High Parameter Discovery канала FlowJo Media
In this webinar Application Scientist, Dr. Timothy Quinn Crawford explores Dimensionality Reduction and Clustering through FlowJo and SeqGeq plugins.
Dimensionality Reduction has become a popular way of visualizing high parameter datasets and is useful in overcoming some of the major obstacles associated with this type of data. The goal in dimensionality reduction is to reduce the number of variables under consideration (i.e., gene reads) and to obtain a set of principal variables (i.e., analytical parameters). This is particularly useful as working with too many dimensions would be overwhelming and one needs some help to visualize this amount of information. Dimensionality reduction takes your high dimensional dataset and allows researchers to view it on a biaxial plot while preserving variance contained in biological populations.
You can download plugins for yourself on FlowJo Exchange: https://www.flowjo.com/exchange/#/
Видео Dimensionality Reduction and Clustering Tools for High Parameter Discovery канала FlowJo Media
Показать
Комментарии отсутствуют
Информация о видео
Другие видео канала
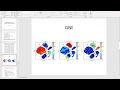
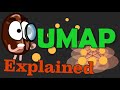
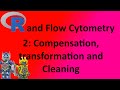
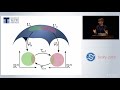
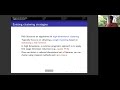
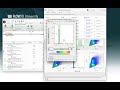
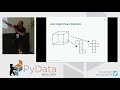


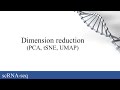
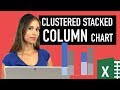
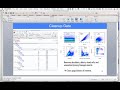
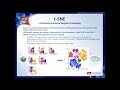
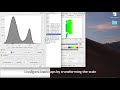

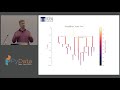
![FlowJo [tSNE]](https://i.ytimg.com/vi/UK725D14-bo/default.jpg)
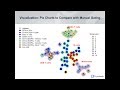
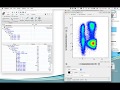
