Modular Language Models
Conventional language models (LMs) are trained densely: all parameters are updated with respect to all data. We argue that dense training leads to a variety of well-documented issues with LMs, including their prohibitive training cost and unreliable downstream behavior. We then introduce a new class of LMs that are fundamentally modular, where components (or experts) of the LM are specialized to distinct domains in the training corpus, and experts are conditionally updated based on the domain of the incoming document. We show how modularity addresses the limitations of dense training by enabling LMs that are rapidly customizable (with the ability to mix, add, or remove experts after training), embarrassingly parallel (requiring no communication between experts), and sparse (needing only a few experts active at a time for inference). Key to our proposal is exploring what constitutes the domains to which experts specialize, as well as reflecting on the data sources used to train LMs. Our new techniques chart a path towards collaborative and personalized LMs, where anyone can contribute to, maintain, and deploy experts at very modest computational cost.
Suchin Gururangan is a 4th year PhD candidate at the University of Washington, advised by Noah A. Smith and Luke Zettlemoyer. He was previously a visiting researcher at Meta AI, a pre-doctoral resident at the Allen Institute for AI, and spent several years in industry as a data scientist. His research interests span many areas of NLP; currently he works on modular, sparse language models that are efficient to customize and scale. His work has received awards at ACL 2020 and 2021, and he is supported by the Bloomberg Data Science PhD Fellowship.
Видео Modular Language Models канала Allen Institute for AI
Suchin Gururangan is a 4th year PhD candidate at the University of Washington, advised by Noah A. Smith and Luke Zettlemoyer. He was previously a visiting researcher at Meta AI, a pre-doctoral resident at the Allen Institute for AI, and spent several years in industry as a data scientist. His research interests span many areas of NLP; currently he works on modular, sparse language models that are efficient to customize and scale. His work has received awards at ACL 2020 and 2021, and he is supported by the Bloomberg Data Science PhD Fellowship.
Видео Modular Language Models канала Allen Institute for AI
Показать
Комментарии отсутствуют
Информация о видео
Другие видео канала
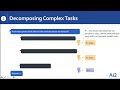
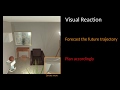
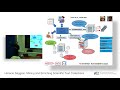
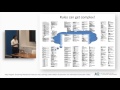

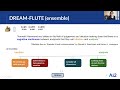
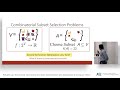
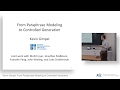
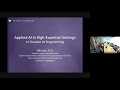
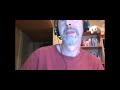
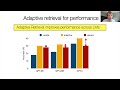
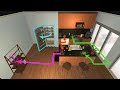

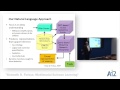
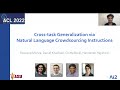
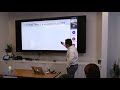
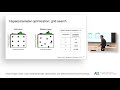
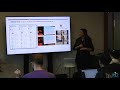
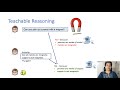
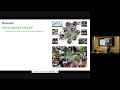
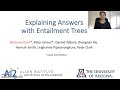