Nathaniel Trask - Structure preservation and convergence in scientific machine learning
Presentation given by Nathaniel Trask on March 3rd 2021 in the one world seminar on the mathematics of machine learning on the topic "Structure preservation and convergence in scientific machine learning ".
Abstract: Physics-informed techniques have emerged as a means of incorporating prior knowledge into machine learning. These techniques generally function by minimizing a hybrid loss, regularizing a traditional $\ell_2$ error with a PDE residual. While remarkably effective, these approaches suffer two major shortcomings. Firstly, such neural network (NN) solutions of PDEs generally fail to converge with increasing architecture size. Despite recent work establishing NNs may approximate at least as well as hp-finite element spaces, in practice when training with gradient methods O(1) optimization errors prevent realizing consistency. Secondly, the regularized losses introduce physics via a penalized residual, and it is well known from classical numerical analysis that the approximation space must be designed in tandem with the residual to ensure converge to a given PDE.
We conjecture that the same tools used to design convergent and structure-preserving properties in forward simulation may be used to design scientific ML architectures with similar guarantees. In this talk, we present two current works which address each of these issues. First, we introduce partition of unity networks (POUnets) to develop convergent approximation with deep networks. It has been shown that traditional feed forward networks may approximate by emulating partitions of unity (POU), and then emulating monomials on each partition, ultimately yielding a localized polynomial approximation and associated hp-convergence. Rather than emulating these components, POUnets function by directly incorporating both the POU and polynomials into the architecture. The resulting approximation breaks the curse of dimensionality and admits a fast least-squares optimization strategy. Predictions are competitive with high-order finite element spaces, and provide superior approximation for problems with reduced regularity.
Secondly, we introduce a data-driven exterior calculus (DDEC) which may be used to endow scientific ML architectures with the structure-preserving properties of mimetic PDE discretization. Traditional mimetic methods function by exploiting the exterior calculus structures offered by a mesh to construct discrete operators that exactly mimic the topological properties of continuum operators. We show how graphs may be used as a surrogate for the topology offered by graphs, and present new network architectures which allows "physics-informed" machine learning which exactly preserves conservation, guarantees extraction of well-posed problems, and allows handling of the non-trivial null-spaces occurring in fields such as electromagnetics.
If time permits, we will additionally share some current results applying these tools in challenging data-driven modeling effort at Sandia, related to data-driven shock hydrodynamics in metals and discovery of surrogates for semiconductors in radiation environments.
Видео Nathaniel Trask - Structure preservation and convergence in scientific machine learning канала One world theoretical machine learning
Abstract: Physics-informed techniques have emerged as a means of incorporating prior knowledge into machine learning. These techniques generally function by minimizing a hybrid loss, regularizing a traditional $\ell_2$ error with a PDE residual. While remarkably effective, these approaches suffer two major shortcomings. Firstly, such neural network (NN) solutions of PDEs generally fail to converge with increasing architecture size. Despite recent work establishing NNs may approximate at least as well as hp-finite element spaces, in practice when training with gradient methods O(1) optimization errors prevent realizing consistency. Secondly, the regularized losses introduce physics via a penalized residual, and it is well known from classical numerical analysis that the approximation space must be designed in tandem with the residual to ensure converge to a given PDE.
We conjecture that the same tools used to design convergent and structure-preserving properties in forward simulation may be used to design scientific ML architectures with similar guarantees. In this talk, we present two current works which address each of these issues. First, we introduce partition of unity networks (POUnets) to develop convergent approximation with deep networks. It has been shown that traditional feed forward networks may approximate by emulating partitions of unity (POU), and then emulating monomials on each partition, ultimately yielding a localized polynomial approximation and associated hp-convergence. Rather than emulating these components, POUnets function by directly incorporating both the POU and polynomials into the architecture. The resulting approximation breaks the curse of dimensionality and admits a fast least-squares optimization strategy. Predictions are competitive with high-order finite element spaces, and provide superior approximation for problems with reduced regularity.
Secondly, we introduce a data-driven exterior calculus (DDEC) which may be used to endow scientific ML architectures with the structure-preserving properties of mimetic PDE discretization. Traditional mimetic methods function by exploiting the exterior calculus structures offered by a mesh to construct discrete operators that exactly mimic the topological properties of continuum operators. We show how graphs may be used as a surrogate for the topology offered by graphs, and present new network architectures which allows "physics-informed" machine learning which exactly preserves conservation, guarantees extraction of well-posed problems, and allows handling of the non-trivial null-spaces occurring in fields such as electromagnetics.
If time permits, we will additionally share some current results applying these tools in challenging data-driven modeling effort at Sandia, related to data-driven shock hydrodynamics in metals and discovery of surrogates for semiconductors in radiation environments.
Видео Nathaniel Trask - Structure preservation and convergence in scientific machine learning канала One world theoretical machine learning
Показать
Комментарии отсутствуют
Информация о видео
4 марта 2021 г. 6:04:05
00:49:41
Другие видео канала
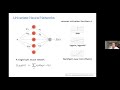
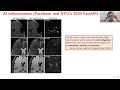

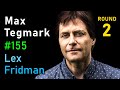
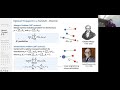
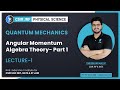
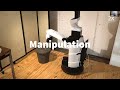
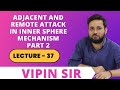
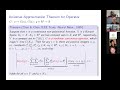
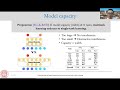
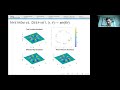
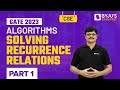
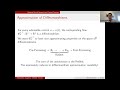
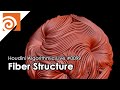
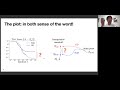
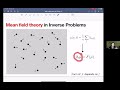
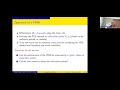
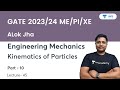
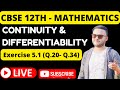
