DDPS | Learning physical simulation with graph network
Talk Abstract
While machine learning models promise to significantly speed up numerical simulations, they are often only accurate in a very narrow range around the training data. In this talk we will present our recent work on neural graph architectures for learning physical dynamics end-to-end. Compared to prevailing CNN architectures, we can demonstrate much stronger generalization and stability properties, and even allows us to simulate larger and more complex setups than seen during training. We will explain the core ideas behind our models, and demonstrate several examples of learned particle and mesh-based simulation on a variety of domains, from aerodynamics over structural mechanics to cloth.
Speaker Biography
Alvaro Sanchez-Gonzalez is a research engineer at DeepMind focused on applying graph neural networks to physical simulation and reinforcement learning. Alvaro has background in Physics (BSc+MSc) and Computer Engineering (BSc), and got his PhD at Imperial College London in 2017 in experimental ultrafast physics using x-ray free electron lasers (XFEL), including applying machine learning to improve XFEL diagnostics.
LLNL-VIDEO-822882
Видео DDPS | Learning physical simulation with graph network канала Livermore Lab Events
While machine learning models promise to significantly speed up numerical simulations, they are often only accurate in a very narrow range around the training data. In this talk we will present our recent work on neural graph architectures for learning physical dynamics end-to-end. Compared to prevailing CNN architectures, we can demonstrate much stronger generalization and stability properties, and even allows us to simulate larger and more complex setups than seen during training. We will explain the core ideas behind our models, and demonstrate several examples of learned particle and mesh-based simulation on a variety of domains, from aerodynamics over structural mechanics to cloth.
Speaker Biography
Alvaro Sanchez-Gonzalez is a research engineer at DeepMind focused on applying graph neural networks to physical simulation and reinforcement learning. Alvaro has background in Physics (BSc+MSc) and Computer Engineering (BSc), and got his PhD at Imperial College London in 2017 in experimental ultrafast physics using x-ray free electron lasers (XFEL), including applying machine learning to improve XFEL diagnostics.
LLNL-VIDEO-822882
Видео DDPS | Learning physical simulation with graph network канала Livermore Lab Events
Показать
Комментарии отсутствуют
Информация о видео
Другие видео канала
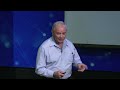
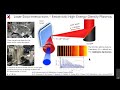


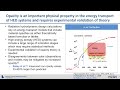

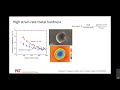
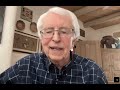
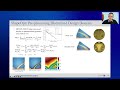
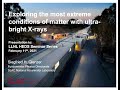
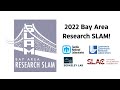


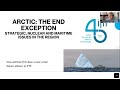
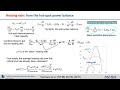
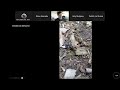
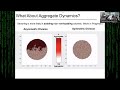
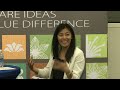

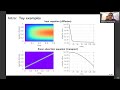
