DDPS | Cheap and robust adaptive reduced order models for nonlinear inversion and design
Description: Nonlinear inverse problems and other PDE-constrained optimization problems, such as structural design under many load cases, require the repeated solution of many discretized large linear systems (or nonlinear systems). For Newton-type methods we also need solutions for the adjoint problem or transpose system. This leads to very high computational costs. These high computational costs can be substantially reduced by using parametric model reduction or other surrogate models as well as by randomization. However, while parametric model reduction is very effective, the online computation of reduced models itself is quite expensive for these problems; moreover, parametric reduced order models do not come with (cheap) error bounds. In this talk, we discuss combinations of model reduction and randomization to reduce costs further. We can use randomization to compute a parametric reduced order model at low cost as well as efficiently monitor the accuracy of the reduced order model during the optimization or directly monitor the difference between the reduced model and full model objective function improvement, which is important for trust-region methods. The latter could also be useful for other surrogate models that do not have an easy error bound available. As we want to reduce the cost of computing reduced order models, we also propose an efficient method for updating a reduced order model in the course of the optimization when needed. This is joint work with Selin Aslan, Drayton Munster, and Serkan Gugercin.
Bio: Eric de Sturler is a Professor of Mathematics at Virginia Tech as well as a Professor in the Computational Modeling and Data Analytics program. He was head of the Mathematics department from August 2018 till August 2022. Previously, he was a Professor of Computer Science at the University of Illinois at Urbana-Champaign and a senior research scientist at the Interdisciplinary Project Center for Supercomputing at ETH Zurich. He works on the theory and development of iterative methods for large linear and nonlinear problems, including high performance computing implementations, randomization, optimization, inverse problems, and their applications. He is a Leslie Fox prizewinner. His research is highly interdisciplinary, including collaborations on the optimal design of structures and other computational mechanics problems, tomography and image reconstruction, electronic structure computations, photosynthesis and optimizing plants for carbon fixation, and computer graphics. His work has been supported by major grants from NSF, DOE, NASA, AFOSR, and Sandia National Labs. He has served as an editor for multiple journals, among others, for Applied Numerical Mathematics and for SIAM Journal on Numerical Analysis, and he was the Program Director of the SIAM Activity Group on Supercomputing from 2003 to 2006. He currently serves on the Board of Directors of the Computing Research Association.
DDPS webinar: https://www.librom.net/ddps.html
💻 LLNL News: https://www.llnl.gov/news
📲 Instagram: https://www.instagram.com/livermore_lab
🤳 Facebook: https://www.facebook.com/livermore.lab
🐤 Twitter: https://twitter.com/Livermore_Lab
🔔 Subscribe: https://www.youtube.com/c/LivermoreLab
About LLNL: Lawrence Livermore National Laboratory has a mission of strengthening the United States’ security through development and application of world-class science and technology to: 1) enhance the nation’s defense, 2) reduce the global threat from terrorism and weapons of mass destruction, and 3) respond with vision, quality, integrity and technical excellence to scientific issues of national importance. Learn more about LLNL: https://www.llnl.gov/.
LLNL-VIDEO-842033
#LLNL #DataDrivenPhysicalSimulations #LawrenceLivermoreNationalLaboratory
Видео DDPS | Cheap and robust adaptive reduced order models for nonlinear inversion and design канала Inside Livermore Lab
Bio: Eric de Sturler is a Professor of Mathematics at Virginia Tech as well as a Professor in the Computational Modeling and Data Analytics program. He was head of the Mathematics department from August 2018 till August 2022. Previously, he was a Professor of Computer Science at the University of Illinois at Urbana-Champaign and a senior research scientist at the Interdisciplinary Project Center for Supercomputing at ETH Zurich. He works on the theory and development of iterative methods for large linear and nonlinear problems, including high performance computing implementations, randomization, optimization, inverse problems, and their applications. He is a Leslie Fox prizewinner. His research is highly interdisciplinary, including collaborations on the optimal design of structures and other computational mechanics problems, tomography and image reconstruction, electronic structure computations, photosynthesis and optimizing plants for carbon fixation, and computer graphics. His work has been supported by major grants from NSF, DOE, NASA, AFOSR, and Sandia National Labs. He has served as an editor for multiple journals, among others, for Applied Numerical Mathematics and for SIAM Journal on Numerical Analysis, and he was the Program Director of the SIAM Activity Group on Supercomputing from 2003 to 2006. He currently serves on the Board of Directors of the Computing Research Association.
DDPS webinar: https://www.librom.net/ddps.html
💻 LLNL News: https://www.llnl.gov/news
📲 Instagram: https://www.instagram.com/livermore_lab
🤳 Facebook: https://www.facebook.com/livermore.lab
🐤 Twitter: https://twitter.com/Livermore_Lab
🔔 Subscribe: https://www.youtube.com/c/LivermoreLab
About LLNL: Lawrence Livermore National Laboratory has a mission of strengthening the United States’ security through development and application of world-class science and technology to: 1) enhance the nation’s defense, 2) reduce the global threat from terrorism and weapons of mass destruction, and 3) respond with vision, quality, integrity and technical excellence to scientific issues of national importance. Learn more about LLNL: https://www.llnl.gov/.
LLNL-VIDEO-842033
#LLNL #DataDrivenPhysicalSimulations #LawrenceLivermoreNationalLaboratory
Видео DDPS | Cheap and robust adaptive reduced order models for nonlinear inversion and design канала Inside Livermore Lab
Показать
Комментарии отсутствуют
Информация о видео
Другие видео канала
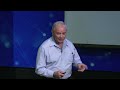
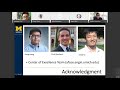

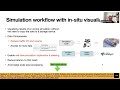

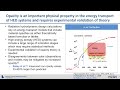
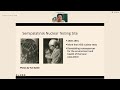

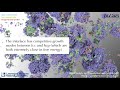
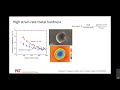
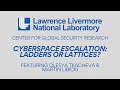
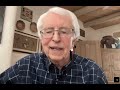
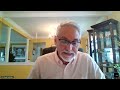
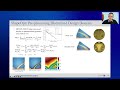
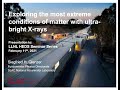
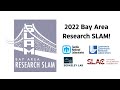


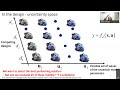
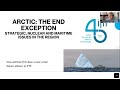