Jan Dubiński - Bucks for buckets (b4b): active defenses against stealing encoders | ML in PL 23
Machine Learning as a Service (MLaaS) APIs provide ready-to-use and high-utility encoders that generate vector representations for given inputs. Since these encoders are very costly to train, they become lucrative targets for model stealing attacks during which an adversary leverages query access to the API to replicate the encoder locally at a fraction of the original training costs. We propose Bucks for Buckets (B4B), the first active defense that prevents stealing while the attack is happening without degrading representation quality for legitimate API users. Our defense relies on the observation that the representations returned to adversaries who try to steal the encoder's functionality cover a significantly larger fraction of the embedding space than representations of legitimate users who utilize the encoder to solve a particular downstream task. B4B leverages this to adaptively adjust the utility of the returned representations according to a user's coverage of the embedding space. To prevent adaptive adversaries from eluding our defense by simply creating multiple user accounts (sybils), B4B also individually transforms each user's representations. This prevents the adversary from directly aggregating representations over multiple accounts to create their stolen encoder copy. Our active defense opens a new path towards securely sharing and democratizing encoders over public APIs.
Jan Dubiński was born in Warsaw, Poland, in 1995. He received a M.Sc. degree in computer science, as well as a B.Sc. and a M.Sc. degrees in power engineering from the Warsaw University of Technology. He also holds a bachelor's degree in quantitative methods from the Warsaw School of Economics, Warsaw. He is currently pursuing a PhD degree in deep learning at the Warsaw University of Technology. He is a member of the ALICE Collaboration at LHC CERN. Jan has been working on fast simulation methods for High Energy Physics experiments at the Large Hadron Collider at CERN. The methods developed in this research leverage generative deep learning models such as GANs to provide a computationally efficient alternative to existing Monte Carlo-based methods. More recently, he has focused on issues related to the security of machine learning models and data privacy. His latest efforts aim to improve the security of self-supervised and generative methods, which are often overlooked compared to supervised models.
The talk was delivered during ML in PL Conference 2023 as a part of Contributed Talks. The conference was organized by a non-profit NGO called ML in PL Association.
ML in PL Association website: https://mlinpl.org/
ML In PL Conference 2023 website: https://conference2023.mlinpl.org/
ML In PL Conference 2024 website: https://conference.mlinpl.org/
---
ML in PL Association was founded based on the experiences in organizing of the ML in PL Conference (formerly PL in ML), the ML in PL Association is a non-profit organization devoted to fostering the machine learning community in Poland and Europe and promoting a deep understanding of ML methods. Even though ML in PL is based in Poland, it seeks to provide opportunities for international cooperation.
Видео Jan Dubiński - Bucks for buckets (b4b): active defenses against stealing encoders | ML in PL 23 канала ML in PL
Jan Dubiński was born in Warsaw, Poland, in 1995. He received a M.Sc. degree in computer science, as well as a B.Sc. and a M.Sc. degrees in power engineering from the Warsaw University of Technology. He also holds a bachelor's degree in quantitative methods from the Warsaw School of Economics, Warsaw. He is currently pursuing a PhD degree in deep learning at the Warsaw University of Technology. He is a member of the ALICE Collaboration at LHC CERN. Jan has been working on fast simulation methods for High Energy Physics experiments at the Large Hadron Collider at CERN. The methods developed in this research leverage generative deep learning models such as GANs to provide a computationally efficient alternative to existing Monte Carlo-based methods. More recently, he has focused on issues related to the security of machine learning models and data privacy. His latest efforts aim to improve the security of self-supervised and generative methods, which are often overlooked compared to supervised models.
The talk was delivered during ML in PL Conference 2023 as a part of Contributed Talks. The conference was organized by a non-profit NGO called ML in PL Association.
ML in PL Association website: https://mlinpl.org/
ML In PL Conference 2023 website: https://conference2023.mlinpl.org/
ML In PL Conference 2024 website: https://conference.mlinpl.org/
---
ML in PL Association was founded based on the experiences in organizing of the ML in PL Conference (formerly PL in ML), the ML in PL Association is a non-profit organization devoted to fostering the machine learning community in Poland and Europe and promoting a deep understanding of ML methods. Even though ML in PL is based in Poland, it seeks to provide opportunities for international cooperation.
Видео Jan Dubiński - Bucks for buckets (b4b): active defenses against stealing encoders | ML in PL 23 канала ML in PL
Показать
Комментарии отсутствуют
Информация о видео
Другие видео канала
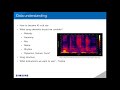
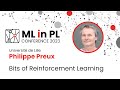
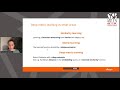
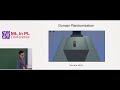
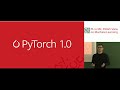

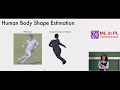
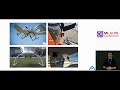
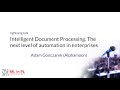
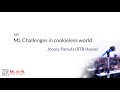
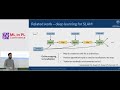
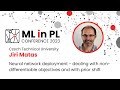
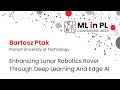


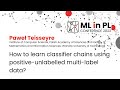
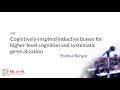

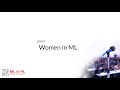
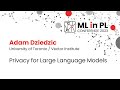
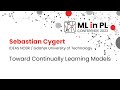