Compliant humanoid robot COMAN learns to walk efficiently
The compliant humanoid robot COMAN learns to walk with two different gaits: one with fixed height of the center of mass, and one with varying height. The varying-height center-of-mass trajectory was learned by reinforcement learning in order to minimize the electric energy consumption during walking. The optimized walking gait achieves 18% reduction of the energy consumption in the sagittal plane, due to the passive compliance - the springs in the knees and ankles of the robot are able to store and release energy efficiently. In addition, the varying-height walking looks more natural and smooth than the conventional fixed-height walking.
This research was presented at the International Conference on Intelligent Robots and Systems (IROS 2011) in September 25-30, 2011 in San Francisco, California.
Video credits:
--------------------------
Dr. Petar Kormushev
http://kormushev.com
Dr. Barkan Ugurlu
Dr. Nikos Tsagarakis
Affiliation:
-------------------------
Department of Advanced Robotics
Italian Institute of Technology
Publication:
---------------------------------
Kormushev, P., Ugurlu, B., Calinon, S., Tsagarakis, N., and Caldwell, D.G., "Bipedal Walking Energy Minimization by Reinforcement Learning with Evolving Policy Parameterization", In Proc. IEEE/RSJ Intl Conf. on Intelligent Robots and Systems (IROS-2011), San Francisco, 2011.
http://kormushev.com/research/publications/
Paper title:
--------------------------
Bipedal Walking Energy Minimization by Reinforcement Learning with Evolving Policy Parameterization
Authors:
---------------------------------
Petar Kormushev, Barkan Ugurlu, Sylvain Calinon, Nikolaos G. Tsagarakis, Darwin G. Caldwell
Paper abstract:
--------------------------
We present a learning-based approach for minimizing the electric energy consumption during walking of a passively-compliant bipedal robot. The energy consumption is reduced by learning a varying-height center-of-mass trajectory which uses efficiently the robot's passive compliance. To do this, we propose a reinforcement learning method which evolves the policy parameterization dynamically during the learning process and thus manages to find better policies faster than by using fixed parameterization. The method is first tested on a function approximation task, and then applied to the humanoid robot COMAN where it achieves significant energy reduction.
Other videos:
-------------------------------------
http://kormushev.com/research/videos/
.
Видео Compliant humanoid robot COMAN learns to walk efficiently канала PetarKormushev
This research was presented at the International Conference on Intelligent Robots and Systems (IROS 2011) in September 25-30, 2011 in San Francisco, California.
Video credits:
--------------------------
Dr. Petar Kormushev
http://kormushev.com
Dr. Barkan Ugurlu
Dr. Nikos Tsagarakis
Affiliation:
-------------------------
Department of Advanced Robotics
Italian Institute of Technology
Publication:
---------------------------------
Kormushev, P., Ugurlu, B., Calinon, S., Tsagarakis, N., and Caldwell, D.G., "Bipedal Walking Energy Minimization by Reinforcement Learning with Evolving Policy Parameterization", In Proc. IEEE/RSJ Intl Conf. on Intelligent Robots and Systems (IROS-2011), San Francisco, 2011.
http://kormushev.com/research/publications/
Paper title:
--------------------------
Bipedal Walking Energy Minimization by Reinforcement Learning with Evolving Policy Parameterization
Authors:
---------------------------------
Petar Kormushev, Barkan Ugurlu, Sylvain Calinon, Nikolaos G. Tsagarakis, Darwin G. Caldwell
Paper abstract:
--------------------------
We present a learning-based approach for minimizing the electric energy consumption during walking of a passively-compliant bipedal robot. The energy consumption is reduced by learning a varying-height center-of-mass trajectory which uses efficiently the robot's passive compliance. To do this, we propose a reinforcement learning method which evolves the policy parameterization dynamically during the learning process and thus manages to find better policies faster than by using fixed parameterization. The method is first tested on a function approximation task, and then applied to the humanoid robot COMAN where it achieves significant energy reduction.
Other videos:
-------------------------------------
http://kormushev.com/research/videos/
.
Видео Compliant humanoid robot COMAN learns to walk efficiently канала PetarKormushev
Показать
Комментарии отсутствуют
Информация о видео
Другие видео канала

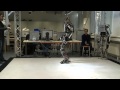

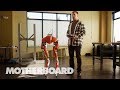
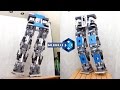
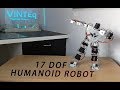
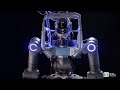
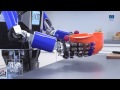
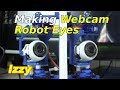
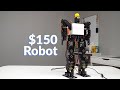
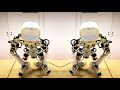
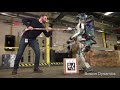

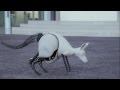
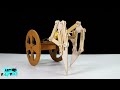

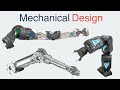
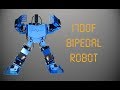
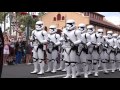
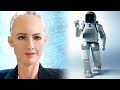