Bhavana Dalvi: Semi Supervised Learning In Realistic Settings
Bhavana Dalvi
"Semi-supervised Learning in Realistic Settings"
05 FEB 2015
Abstract:
Semi-supervised learning (SSL) has been widely used over a decade for various tasks -- including knowledge acquisition-- that lack large amount of training data. My research proposes a novel learning scenario in which the system knows a few categories in advance, but the rest of the categories are unanticipated and need to be discovered from the unlabeled data. With the availability of enormous unlabeled datasets at low cost, and difficulty of collecting labeled data for all possible categories, it becomes even more important to adapt traditional semi-supervised learning techniques to such realistic settings.
In this talk, I will start by discussing three realistic scenarios for SSL: incorporating unanticipated classes, ontological constraints, and multiple data views. Then I will talk about our Exploratory Learning techniques that can jointly learn existing categories and discover new categories from the unlabeled data, thereby reducing semantic drift in traditional SSL. Next, I will talk about our recent work that does SSL by incorporating ontological constraints between categories. I will also demonstrate an application of this method for the task of automatic gloss finding for a knowledge base. Finally, I will conclude the talk with a summary of my other research projects and several directions for future research.
Видео Bhavana Dalvi: Semi Supervised Learning In Realistic Settings канала Allen Institute for AI
"Semi-supervised Learning in Realistic Settings"
05 FEB 2015
Abstract:
Semi-supervised learning (SSL) has been widely used over a decade for various tasks -- including knowledge acquisition-- that lack large amount of training data. My research proposes a novel learning scenario in which the system knows a few categories in advance, but the rest of the categories are unanticipated and need to be discovered from the unlabeled data. With the availability of enormous unlabeled datasets at low cost, and difficulty of collecting labeled data for all possible categories, it becomes even more important to adapt traditional semi-supervised learning techniques to such realistic settings.
In this talk, I will start by discussing three realistic scenarios for SSL: incorporating unanticipated classes, ontological constraints, and multiple data views. Then I will talk about our Exploratory Learning techniques that can jointly learn existing categories and discover new categories from the unlabeled data, thereby reducing semantic drift in traditional SSL. Next, I will talk about our recent work that does SSL by incorporating ontological constraints between categories. I will also demonstrate an application of this method for the task of automatic gloss finding for a knowledge base. Finally, I will conclude the talk with a summary of my other research projects and several directions for future research.
Видео Bhavana Dalvi: Semi Supervised Learning In Realistic Settings канала Allen Institute for AI
Показать
Комментарии отсутствуют
Информация о видео
Другие видео канала
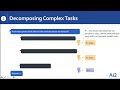
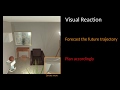
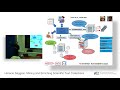
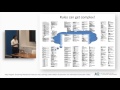

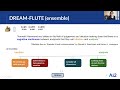
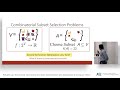
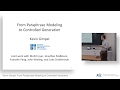
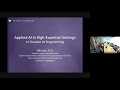
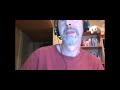
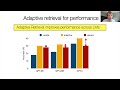
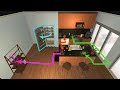

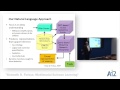
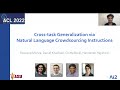
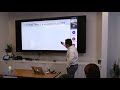
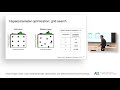
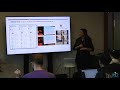
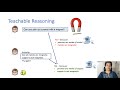
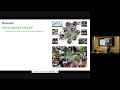
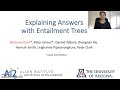