Arvind Neelakantan: Text and Code Embeddings
Text embeddings are useful features in many applications such as semantic search and computing text similarity. Previous work typically trains models customized for different use cases, varying in dataset choice, training objective and model architecture. In this work, Arvind Neelakantan (OpenAI) shows that contrastive pre-training on unsupervised data at scale leads to high quality vector representations of text and code. The same unsupervised text embeddings that achieve new state-of-the-art results in linear-probe classification also display impressive semantic search capabilities and sometimes even perform competitively with fine-tuned models. Similarly to text embeddings, he trains code embedding models on (text, code) pairs, obtaining a 20.8% relative improvement over prior best work on code search.
Видео Arvind Neelakantan: Text and Code Embeddings канала Oxford ML and Physics Seminars
Видео Arvind Neelakantan: Text and Code Embeddings канала Oxford ML and Physics Seminars
Показать
Комментарии отсутствуют
Информация о видео
13 июня 2022 г. 14:00:45
00:36:36
Другие видео канала
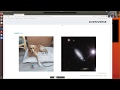
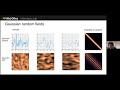
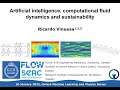
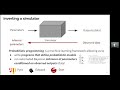
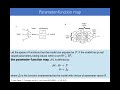



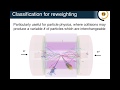
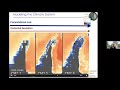
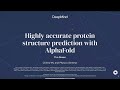
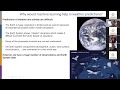
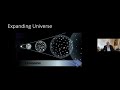
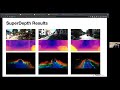


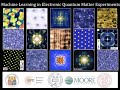
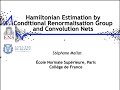
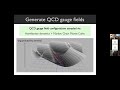
