Atılım Güneş Baydin: Probabilistic Programming for Inverse Problems in the Physical Sciences
Machine learning enables new approaches to inverse problems in many fields of science. We present a novel probabilistic programming framework that couples directly to existing scientific simulators through a cross-platform probabilistic execution protocol, which allows general-purpose inference engines to record and control random number draws within simulators in a language-agnostic way. The execution of existing simulators as probabilistic programs enables highly interpretable posterior inference in the structured model defined by the simulator code base. We demonstrate the technique in particle physics, on a scientifically accurate simulation of the tau lepton decay, which is a key ingredient in establishing the properties of the Higgs boson. Inference efficiency is achieved via amortized inference where a deep recurrent neural network is trained to parameterize proposal distributions and control the stochastic simulator in a sequential importance sampling scheme, at a fraction of the computational cost of a Markov chain Monte Carlo baseline.
Видео Atılım Güneş Baydin: Probabilistic Programming for Inverse Problems in the Physical Sciences канала Oxford ML and Physics Seminars
Видео Atılım Güneş Baydin: Probabilistic Programming for Inverse Problems in the Physical Sciences канала Oxford ML and Physics Seminars
Показать
Комментарии отсутствуют
Информация о видео
21 января 2021 г. 23:14:34
01:11:23
Другие видео канала
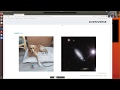

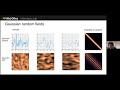
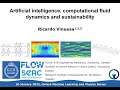
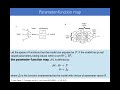



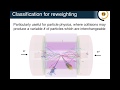
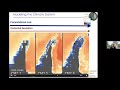
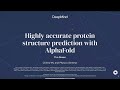
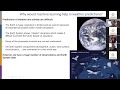
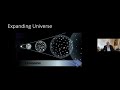
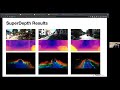


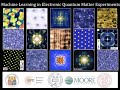
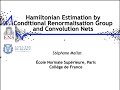
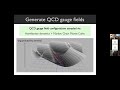
