Best Paper Awards NeurIPS 2018: Non-delusional Q-learning and Value-iteration
Join the channel membership:
https://www.youtube.com/c/AIPursuit/join
Subscribe to the channel:
https://www.youtube.com/c/AIPursuit?sub_confirmation=1
Support and Donation:
Paypal ⇢ https://paypal.me/tayhengee
Patreon ⇢ https://www.patreon.com/hengee
BTC ⇢ bc1q2r7eymlf20576alvcmryn28tgrvxqw5r30cmpu
ETH ⇢ 0x58c4bD4244686F3b4e636EfeBD159258A5513744
Doge ⇢ DSGNbzuS1s6x81ZSbSHHV5uGDxJXePeyKy
-----------------------------------------------------------------------------------------
Credit to Tyler Lu and NeurIPS 2018
-----------------------------------------------------------------------------------------
The paper: https://papers.nips.cc/paper/8200-non-delusional-q-learning-and-value-iteration
The original conference video: https://www.facebook.com/nipsfoundation/videos/357066758186895/
Abstract:
We identify a fundamental source of error in Q-learning and other forms of dynamic programming with function approximation. Delusional bias arises when the approximation architecture limits the class of expressible greedy policies. Since standard Q-updates make globally uncoordinated action choices with respect to the expressible policy class, inconsistent or even conflicting Q-value estimates can result, leading to pathological behaviour such as over/under-estimation, instability and even divergence. To solve this problem, we introduce a new notion of policy consistency and define a local backup process that ensures global consistency through the use of information sets---sets that record constraints on policies consistent with backed-up Q-values. We prove that both the model-based and model-free algorithms using this backup remove delusional bias, yielding the first known algorithms that guarantee optimal results under general conditions. These algorithms furthermore only require polynomially many information sets (from a potentially exponential support). Finally, we suggest other practical heuristics for value-iteration and Q-learning that attempt to reduce delusional bias.
Q-Learning, Deep Reinforcement Learning
Видео Best Paper Awards NeurIPS 2018: Non-delusional Q-learning and Value-iteration канала AI Pursuit by TAIR
https://www.youtube.com/c/AIPursuit/join
Subscribe to the channel:
https://www.youtube.com/c/AIPursuit?sub_confirmation=1
Support and Donation:
Paypal ⇢ https://paypal.me/tayhengee
Patreon ⇢ https://www.patreon.com/hengee
BTC ⇢ bc1q2r7eymlf20576alvcmryn28tgrvxqw5r30cmpu
ETH ⇢ 0x58c4bD4244686F3b4e636EfeBD159258A5513744
Doge ⇢ DSGNbzuS1s6x81ZSbSHHV5uGDxJXePeyKy
-----------------------------------------------------------------------------------------
Credit to Tyler Lu and NeurIPS 2018
-----------------------------------------------------------------------------------------
The paper: https://papers.nips.cc/paper/8200-non-delusional-q-learning-and-value-iteration
The original conference video: https://www.facebook.com/nipsfoundation/videos/357066758186895/
Abstract:
We identify a fundamental source of error in Q-learning and other forms of dynamic programming with function approximation. Delusional bias arises when the approximation architecture limits the class of expressible greedy policies. Since standard Q-updates make globally uncoordinated action choices with respect to the expressible policy class, inconsistent or even conflicting Q-value estimates can result, leading to pathological behaviour such as over/under-estimation, instability and even divergence. To solve this problem, we introduce a new notion of policy consistency and define a local backup process that ensures global consistency through the use of information sets---sets that record constraints on policies consistent with backed-up Q-values. We prove that both the model-based and model-free algorithms using this backup remove delusional bias, yielding the first known algorithms that guarantee optimal results under general conditions. These algorithms furthermore only require polynomially many information sets (from a potentially exponential support). Finally, we suggest other practical heuristics for value-iteration and Q-learning that attempt to reduce delusional bias.
Q-Learning, Deep Reinforcement Learning
Видео Best Paper Awards NeurIPS 2018: Non-delusional Q-learning and Value-iteration канала AI Pursuit by TAIR
Показать
Комментарии отсутствуют
Информация о видео
Другие видео канала


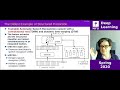

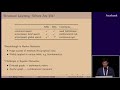
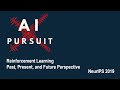
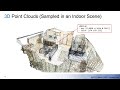
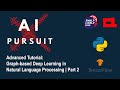
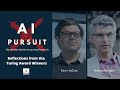
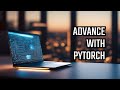
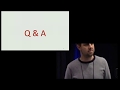
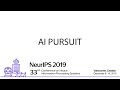
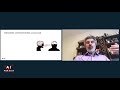
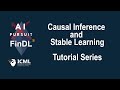
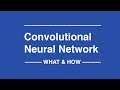
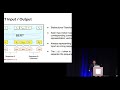
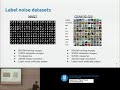
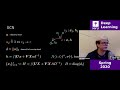
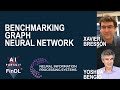
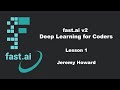
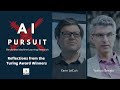