Causal Inference and Stable Learning by Peng Cui
Join the channel membership:
https://www.youtube.com/c/AIPursuit/join
Subscribe to the channel:
https://www.youtube.com/c/AIPursuit?sub_confirmation=1
Support and Donation:
Paypal ⇢ https://paypal.me/tayhengee
Patreon ⇢ https://www.patreon.com/hengee
BTC ⇢ bc1q2r7eymlf20576alvcmryn28tgrvxqw5r30cmpu
ETH ⇢ 0x58c4bD4244686F3b4e636EfeBD159258A5513744
Doge ⇢ DSGNbzuS1s6x81ZSbSHHV5uGDxJXePeyKy
Wanted to own BTC, ETH, or even Dogecoin? Kickstart your crypto portfolio with the largest crypto market Binance with my affiliate link:
https://accounts.binance.com/en/register?ref=27700065
BuyMeACoffee: https://www.buymeacoffee.com/angustay
-----------------------------------------------------------------------------------------
The video is reposted for educational purposes.
Source: https://slideslive.com/38917403/causal-inference-and-stable-learning
Abstract:
Predicting future outcome values based on their observed features using a model estimated on a training data set in a common machine learning problem. Many learning algorithms have been proposed and shown to be successful when the test data and training data come from the same distribution. However, the best-performing models for a given distribution of training data typically exploit subtle statistical relationships among features, making them potentially more prone to prediction error when applied to test data whose distribution differs from that in training data. How to develop learning models that are stable and robust to shifts in data is of paramount importance for both academic research and real applications. Causal inference, which refers to the process of drawing a conclusion about a causal connection based on the conditions of the occurrence of an effect, is a powerful statistical modeling tool for explanatory and stable learning. In this tutorial, we focus on causal inference and stable learning, aiming to explore causal knowledge from observational data to improve the interpretability and stability of machine learning algorithms. First, we will give an introduction to causal inference and introduce some recent data-driven approaches to estimate causal effect from observational data, especially in the high dimensional settings. Aiming to bridge the gap between causal inference and machine learning for stable learning, we first give the definition of stability and robustness of learning algorithms, then will introduce some recently stable learning algorithms for improving the stability and interpretability of prediction. Finally, we will discuss the applications and future directions of stable learning, and provide the benchmark for stable learning.
Видео Causal Inference and Stable Learning by Peng Cui канала DSAI by Dr. Osbert Tay
https://www.youtube.com/c/AIPursuit/join
Subscribe to the channel:
https://www.youtube.com/c/AIPursuit?sub_confirmation=1
Support and Donation:
Paypal ⇢ https://paypal.me/tayhengee
Patreon ⇢ https://www.patreon.com/hengee
BTC ⇢ bc1q2r7eymlf20576alvcmryn28tgrvxqw5r30cmpu
ETH ⇢ 0x58c4bD4244686F3b4e636EfeBD159258A5513744
Doge ⇢ DSGNbzuS1s6x81ZSbSHHV5uGDxJXePeyKy
Wanted to own BTC, ETH, or even Dogecoin? Kickstart your crypto portfolio with the largest crypto market Binance with my affiliate link:
https://accounts.binance.com/en/register?ref=27700065
BuyMeACoffee: https://www.buymeacoffee.com/angustay
-----------------------------------------------------------------------------------------
The video is reposted for educational purposes.
Source: https://slideslive.com/38917403/causal-inference-and-stable-learning
Abstract:
Predicting future outcome values based on their observed features using a model estimated on a training data set in a common machine learning problem. Many learning algorithms have been proposed and shown to be successful when the test data and training data come from the same distribution. However, the best-performing models for a given distribution of training data typically exploit subtle statistical relationships among features, making them potentially more prone to prediction error when applied to test data whose distribution differs from that in training data. How to develop learning models that are stable and robust to shifts in data is of paramount importance for both academic research and real applications. Causal inference, which refers to the process of drawing a conclusion about a causal connection based on the conditions of the occurrence of an effect, is a powerful statistical modeling tool for explanatory and stable learning. In this tutorial, we focus on causal inference and stable learning, aiming to explore causal knowledge from observational data to improve the interpretability and stability of machine learning algorithms. First, we will give an introduction to causal inference and introduce some recent data-driven approaches to estimate causal effect from observational data, especially in the high dimensional settings. Aiming to bridge the gap between causal inference and machine learning for stable learning, we first give the definition of stability and robustness of learning algorithms, then will introduce some recently stable learning algorithms for improving the stability and interpretability of prediction. Finally, we will discuss the applications and future directions of stable learning, and provide the benchmark for stable learning.
Видео Causal Inference and Stable Learning by Peng Cui канала DSAI by Dr. Osbert Tay
Показать
Комментарии отсутствуют
Информация о видео
Другие видео канала


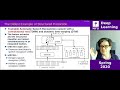

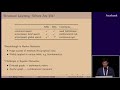
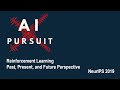
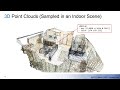
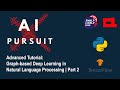
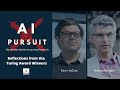
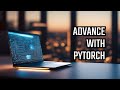
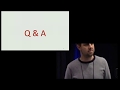
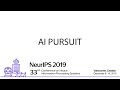
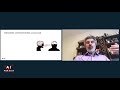
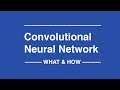
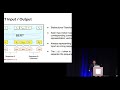
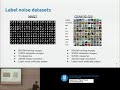
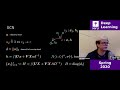
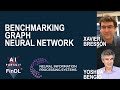
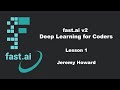
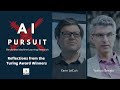