Forecasting Crop Productivity with High-Resolution Satellite Data: Scaling Up to the Whole...
"Forecasting Crop Productivity with High-Resolution Satellite Data: Scaling Up to the Whole US Corn Belt" -- Sibo Wang, University of Illinois at Urbana-Champaign
High-performance computing, along with satellite datasets that provide a wide spatiotemporal coverage of agricultural lands, enables a novel and data-oriented approach to understand crop growth. Using Blue Waters, we developed a hybrid crop model (CLM-APSIM) that produces reliable long-term yield predictions in the US Corn Belt. We also developed a generic multi-sensor fusion algorithm, STAIR, that integrates satellite images at a variety of spatial and temporal resolutions. Finally, a pixel-level crop yield model, ASPIRE, uses remotely sensed images, soil condition, and climate data to predict crop yield at field level. The ultimate goal of our project is to improve our predictability skill for global crop yield modeling by integrating site measurements, satellite observations, and process-based modeling.
Видео Forecasting Crop Productivity with High-Resolution Satellite Data: Scaling Up to the Whole... канала NCSAatIllinois
High-performance computing, along with satellite datasets that provide a wide spatiotemporal coverage of agricultural lands, enables a novel and data-oriented approach to understand crop growth. Using Blue Waters, we developed a hybrid crop model (CLM-APSIM) that produces reliable long-term yield predictions in the US Corn Belt. We also developed a generic multi-sensor fusion algorithm, STAIR, that integrates satellite images at a variety of spatial and temporal resolutions. Finally, a pixel-level crop yield model, ASPIRE, uses remotely sensed images, soil condition, and climate data to predict crop yield at field level. The ultimate goal of our project is to improve our predictability skill for global crop yield modeling by integrating site measurements, satellite observations, and process-based modeling.
Видео Forecasting Crop Productivity with High-Resolution Satellite Data: Scaling Up to the Whole... канала NCSAatIllinois
Показать
Комментарии отсутствуют
Информация о видео
Другие видео канала

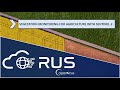
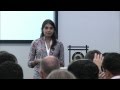

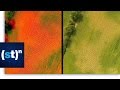
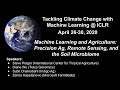
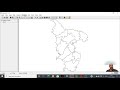


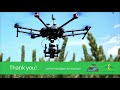
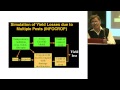
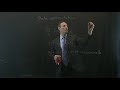
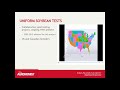
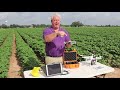
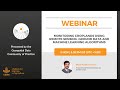
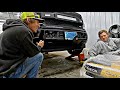

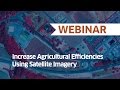
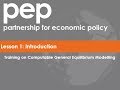
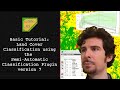