Attacking Optical Flow
Keynote presented on June 14, 2020 at CVPR in the
SAIAD - Safe Artificial Intelligence for Automated Driving Workshop
Slides: http://www.cvlibs.net/talks/talk_cvpr_2020_flow_attack.pdf
Paper: http://www.cvlibs.net/publications/Ranjan2019ICCV.pdf
Abstract: Deep neural nets achieve state-of-the-art performance on the problem of optical flow estimation. Since optical flow is used in several safety-critical applications like self-driving cars, it is important to gain insights into the robustness of those techniques. Recently, it has been shown that adversarial attacks easily fool deep neural networks to misclassify objects. The robustness of optical flow networks to adversarial attacks, however, has not been studied so far. In this talk, I will extend adversarial patch attacks to optical flow networks and show that such attacks can compromise their performance. I will demonstrate that corrupting a small patch of less than 1% of the image size can significantly affect optical flow estimates. The resulting attacks lead to noisy flow estimates that extend significantly beyond the region of the attack. I will show that networks using an encoder-decoder architecture are very sensitive to these attacks while networks using a spatial pyramid architecture are less affected.
Видео Attacking Optical Flow канала Andreas Geiger
SAIAD - Safe Artificial Intelligence for Automated Driving Workshop
Slides: http://www.cvlibs.net/talks/talk_cvpr_2020_flow_attack.pdf
Paper: http://www.cvlibs.net/publications/Ranjan2019ICCV.pdf
Abstract: Deep neural nets achieve state-of-the-art performance on the problem of optical flow estimation. Since optical flow is used in several safety-critical applications like self-driving cars, it is important to gain insights into the robustness of those techniques. Recently, it has been shown that adversarial attacks easily fool deep neural networks to misclassify objects. The robustness of optical flow networks to adversarial attacks, however, has not been studied so far. In this talk, I will extend adversarial patch attacks to optical flow networks and show that such attacks can compromise their performance. I will demonstrate that corrupting a small patch of less than 1% of the image size can significantly affect optical flow estimates. The resulting attacks lead to noisy flow estimates that extend significantly beyond the region of the attack. I will show that networks using an encoder-decoder architecture are very sensitive to these attacks while networks using a spatial pyramid architecture are less affected.
Видео Attacking Optical Flow канала Andreas Geiger
Показать
Комментарии отсутствуют
Информация о видео
Другие видео канала

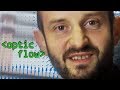
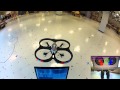
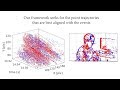
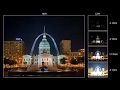
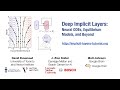
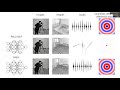
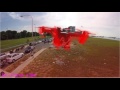
![[CVPR 2020] Self-Supervised Monocular Scene Flow Estimation (5-min talk)](https://i.ytimg.com/vi/1lR6PQO82lc/default.jpg)
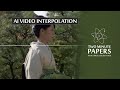
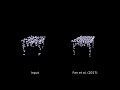
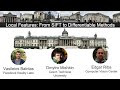
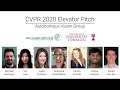


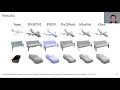
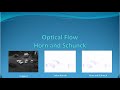
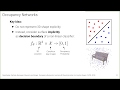

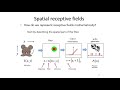