Learning 3D Reconstruction in Function Space (Long Version)
Keynote presented on June 19, 2020 at CVPR in the
Joint Workshop on Deep Learning Foundations of Geometric Shape Modeling and Reconstruction
Slides: http://www.cvlibs.net/talks/talk_cvpr_2020_implicit_long.pdf
Papers:
http://www.cvlibs.net/publications/Mescheder2019CVPR.pdf
http://www.cvlibs.net/publications/Oechsle2019ICCV.pdf
http://www.cvlibs.net/publications/Niemeyer2019ICCV.pdf
http://www.cvlibs.net/publications/Niemeyer2020CVPR.pdf
Abstract: I will show several recent results on learning neural implicit 3D representations by departing from the traditional concept of representing 3D shapes explicitly using voxels, points and meshes. Implicit representations have a small memory footprint and allow for modeling arbitrary 3D toplogies at arbitrary resolution in continuous function space. I will show the abilitiy and limitations of these approaches in the context of reconstructing 3D geometry and textured 3D models and motion. Finally, I will also show results on learning implicit 3D models using only 2D supervision by deriving an analytic closed form solution to the gradient updates.
Видео Learning 3D Reconstruction in Function Space (Long Version) канала Andreas Geiger
Joint Workshop on Deep Learning Foundations of Geometric Shape Modeling and Reconstruction
Slides: http://www.cvlibs.net/talks/talk_cvpr_2020_implicit_long.pdf
Papers:
http://www.cvlibs.net/publications/Mescheder2019CVPR.pdf
http://www.cvlibs.net/publications/Oechsle2019ICCV.pdf
http://www.cvlibs.net/publications/Niemeyer2019ICCV.pdf
http://www.cvlibs.net/publications/Niemeyer2020CVPR.pdf
Abstract: I will show several recent results on learning neural implicit 3D representations by departing from the traditional concept of representing 3D shapes explicitly using voxels, points and meshes. Implicit representations have a small memory footprint and allow for modeling arbitrary 3D toplogies at arbitrary resolution in continuous function space. I will show the abilitiy and limitations of these approaches in the context of reconstructing 3D geometry and textured 3D models and motion. Finally, I will also show results on learning implicit 3D models using only 2D supervision by deriving an analytic closed form solution to the gradient updates.
Видео Learning 3D Reconstruction in Function Space (Long Version) канала Andreas Geiger
Показать
Комментарии отсутствуют
Информация о видео
Другие видео канала
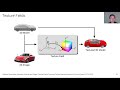
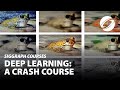
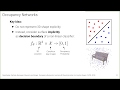
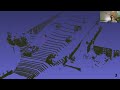
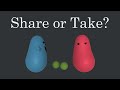
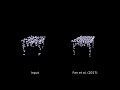
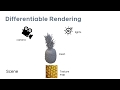
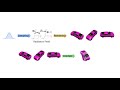
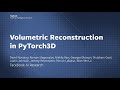
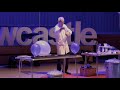
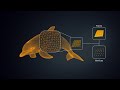
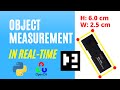
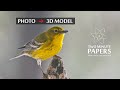
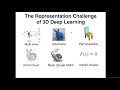


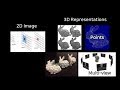
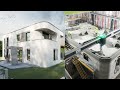
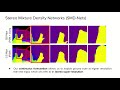
