On Parameter Efficiency of Neural Language Models
Abstract: Pre-trained neural language models have demonstrated remarkable generalizability in various downstream tasks, such as natural language understanding and question answering. However, these models have grown to contain hundreds of billions of parameters, making them difficult to be deployed in applications with latency requirements and memory constraints. Furthermore, existing research have demonstrated the existence of significant redundant parameters in neural language models. Such redundancy can further compromise their downstream generalizability. To tackle these challenges, my research focus on training neural language models towards higher parameter efficiency and better model generalizability. In this talk, I will introduce three major directions of my research: 1) designing model training algorithms for better parameter utilization, 2) developing pruning and distillation strategies for reliable model compression, and 3) improving cross-task generalizability of parameter-efficient fine-tuning methods.
Bio: Chen Liang is a final-year PhD student from Georgia Institute of Technology, working with Prof. Tuo Zhao in the FLASH research group. Her research interests broadly lie in deep learning and natural language processing, with a major focus on developing methodologies and algorithms to improve model generalizability and parameter efficiency for pre-trained neural language models. Before starting her PhD, Chen received her BS degree in Electrical Engineering from University of Southern California.
link: https://cliang1453.github.io/
Видео On Parameter Efficiency of Neural Language Models канала Allen Institute for AI
Bio: Chen Liang is a final-year PhD student from Georgia Institute of Technology, working with Prof. Tuo Zhao in the FLASH research group. Her research interests broadly lie in deep learning and natural language processing, with a major focus on developing methodologies and algorithms to improve model generalizability and parameter efficiency for pre-trained neural language models. Before starting her PhD, Chen received her BS degree in Electrical Engineering from University of Southern California.
link: https://cliang1453.github.io/
Видео On Parameter Efficiency of Neural Language Models канала Allen Institute for AI
Показать
Комментарии отсутствуют
Информация о видео
Другие видео канала
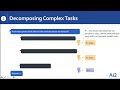
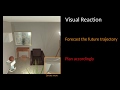
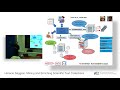
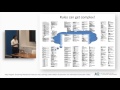

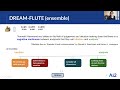
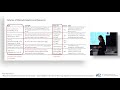
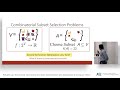
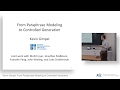
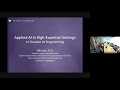
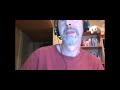
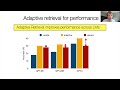
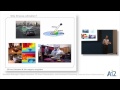
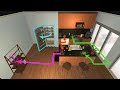

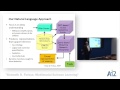
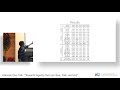
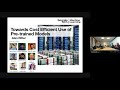
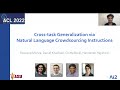
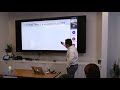
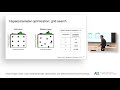