Keynote 1: High dimensional Causal Inference -- Peter Bühlman
Understanding cause-effect relationships between variables is of great interest in many fields of science. An ambitious but highly desirable goal is to infer causal effects from observational data obtained by observing a system of interest without subjecting it to interventions. This would allow to circumvent severe experimental constraints or to substantially lower experimental costs. Our main motivation to study this goal comes from applications in biology.
We present recent progress for estimating causal graphs (networks) and prediction of causal effects. Various restrictions in structural equation models lead to better or full identifiability, and statistical estimation and inference can be done using order search and penalized (sparse) regression. The methods and algorithms are computationally feasible and statistically consistent, even for high-dimensional settings with thousands of variables but a few observations only. We highlight exciting possibilities and fundamental limitations. In view of the latter, statistical modeling should be complemented with experimental validations: we discuss this in the context of molecular biology for yeast (Saccharomyces Cerevisiae) and the model plant Arabidopsis Thaliana.
Видео Keynote 1: High dimensional Causal Inference -- Peter Bühlman канала AISTATS 2014
We present recent progress for estimating causal graphs (networks) and prediction of causal effects. Various restrictions in structural equation models lead to better or full identifiability, and statistical estimation and inference can be done using order search and penalized (sparse) regression. The methods and algorithms are computationally feasible and statistically consistent, even for high-dimensional settings with thousands of variables but a few observations only. We highlight exciting possibilities and fundamental limitations. In view of the latter, statistical modeling should be complemented with experimental validations: we discuss this in the context of molecular biology for yeast (Saccharomyces Cerevisiae) and the model plant Arabidopsis Thaliana.
Видео Keynote 1: High dimensional Causal Inference -- Peter Bühlman канала AISTATS 2014
Показать
Комментарии отсутствуют
Информация о видео
Другие видео канала
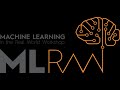
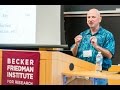
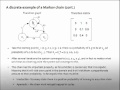
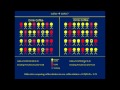
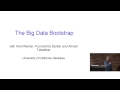

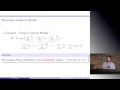
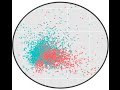
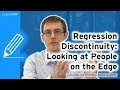
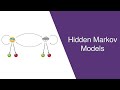
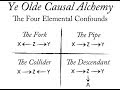
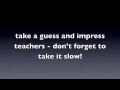

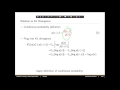
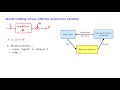
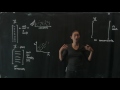
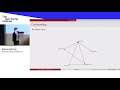
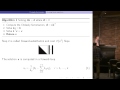
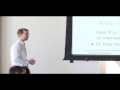
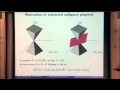