Unifying the Counterfactual and Graphical Approaches to Causality
Models based on potential outcomes, also known as counterfactuals, were introduced by Neyman (1923) and later applied to observational contexts by Rubin (1974). Such models are now used extensively within Biostatistics, Statistics, Political Science, Economics, and Epidemiology for reasoning about causation. Directed acyclic graphs (DAGs), introduced by Wright (1921) are another formalism used to represent causal systems. Graphs are also extensively used in Computer Science, Bioinformatics, Sociology and Epidemiology.
I present a simple approach to unifying these two frameworks via a new graph, termed the Single-World Intervention Graph (SWIG). The SWIG encodes the counterfactual independences associated with a specific hypothetical intervention on a set of treatment variables. The nodes on the SWIG are the corresponding counterfactual random variables. The SWIG is derived from a causal DAG via a simple node splitting transformation. I will illustrate the theory with a number of examples. Finally I show that SWIGs avoid a number of pitfalls that are present in an alternative approach to unification, based on `twin networks' that has been advocated by Pearl (2000).
Видео Unifying the Counterfactual and Graphical Approaches to Causality канала Fung Institute for Engineering Leadership
I present a simple approach to unifying these two frameworks via a new graph, termed the Single-World Intervention Graph (SWIG). The SWIG encodes the counterfactual independences associated with a specific hypothetical intervention on a set of treatment variables. The nodes on the SWIG are the corresponding counterfactual random variables. The SWIG is derived from a causal DAG via a simple node splitting transformation. I will illustrate the theory with a number of examples. Finally I show that SWIGs avoid a number of pitfalls that are present in an alternative approach to unification, based on `twin networks' that has been advocated by Pearl (2000).
Видео Unifying the Counterfactual and Graphical Approaches to Causality канала Fung Institute for Engineering Leadership
Показать
Комментарии отсутствуют
Информация о видео
20 марта 2014 г. 23:47:52
01:24:07
Другие видео канала
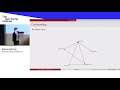
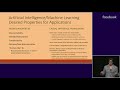
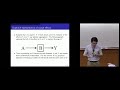
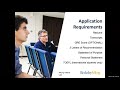
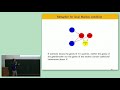
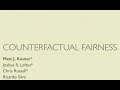
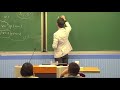
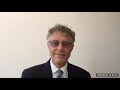
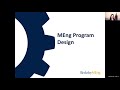
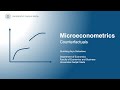
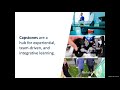
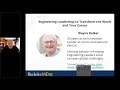
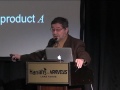
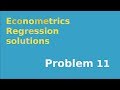
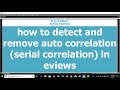
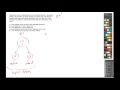
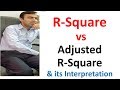